Keeping Skynet at bay: How humans can keep AI in check
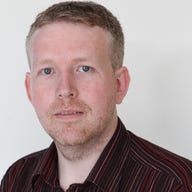
Scientists and investors in the field of artificial intelligence have set out safeguards that may be necessary to control AIs whose capabilities far surpass humans.
The open letter and research from the Future of Life Institute (FLI) explores possible ways of preventing these superintelligent AIs from acting in undesirable and potentially destructive ways.
In its research the institute - whose scientific advisory board includes SpaceX and Tesla co-founder Elon Musk and the physicist Stephen Hawking - says the probability of such intelligences being created is such that the risks need to be considered now.
"To justify a modest investment in this AI robustness research, this probability need not be high, merely non-negligible, just as a modest investment in home insurance is justified by a non-negligible probability of the home burning down," it says, pointing out that in the 1930s one of the greatest physicists of the time, Ernest Rutherford, said nuclear energy was "moonshine", just five years before the discovery of nuclear fission.
"There is now a broad consensus that AI research is progressing steadily, and that its impact on society is likely to increase," it says, citing recent successes in AI-related fields such as speech recognition, image classification, autonomous vehicles, machine translation, walking robots, and question-answering systems.
In light of this progress, the FLI's research paper sets out key areas of research that could help ensure that AI, both weak and strong, is "robust and beneficial" to society.
Checking undesirable behaviour
The problem of defining what an AI should and shouldn't do can be particularly thorny.
For example, take a simple AI agent whose behaviour is governed by the maxim: 'If environment satisfies assumptions x then behaviour should satisfy requirements y'.
Properly defining the required behaviour and outcomes is key, as in attempting to satisfy requirement y it is possible the agent may behave in an undesirable way.
"If a robot vacuum cleaner is asked to clean up as much dirt as possible, and has an action to dump the contents of its dirt container, it will repeatedly dump and clean up the same dirt. The requirement should focus not on dirt cleaned up but on cleanliness of the floor," the report states.
"In order to build systems that robustly behave well, we of course need to decide what 'good behavior' means in each application domain. Designing simplified rules - for example, to govern a self-driving car's decisions in critical situations - will likely require expertise from both ethicists and computer scientists," the report says.
Ensuring proper behaviour becomes even more problematic with strong, general AI, the paper says.
Societies are likely to encounter significant challenges in "aligning" the values of powerful AI systems with their own values and preferences.
"Consider, for instance, the difficulty of creating a utility function that encompasses an entire body of law; even a literal rendition of the law is far beyond our current capabilities, and would be highly unsatisfactory in practice," it states.
Another issue comes from reinforcing and rewarding behaviours in learning machines to achieve a particular outcome. Once the machine becomes generally capable the desire to hit these targets may distort its behaviour, as Goodheart's Law recognises in humans.
Checking for bugs
Just as an airplane's onboard software undergoes rigorous checks for bugs that might trigger unexpected behaviour, so the code that underlies AIs should be subject to similar formal constraints.
For traditional software there are projects such as seL4, which has developed a complete, general-purpose operating-system kernel that has been mathematically checked against a formal specification to give a strong guarantee against crashes and unsafe operations.
However, in the case of AI, new approaches to verification may be needed, according to the FLI.
"Perhaps the most salient difference between verification of traditional software and verification of AI systems is that the correctness of traditional software is defined with respect to a fixed and known machine model, whereas AI systems - especially robots and other embodied systems - operate in environments that are at best partially known by the system designer.
"In these cases, it may be practical to verify that the system acts correctly given the knowledge that it has, avoiding the problem of modelling the real environment," the research states.
The FLI suggests it should be possible to build AI systems from components, each of which has been verified.
"If the theory of extending verifiable properties from components to entire systems is well understood, then even very large systems can enjoy certain kinds of safety guarantees, potentially aided by techniques designed explicitly to handle learning agents and high-level properties."
However, trying to apply formal validation tools to "systems that modify, extend, or improve themselves, possibly many times in succession", is a challenge whose solution is not yet clear, according to the research.
Constraining AI's capabilities
With cyberwarfare likely to play an increasing role in conflicts between nations, the paper sees AI helping to both secure and attack systems.
"It is unclear whether long-term progress in AI will make the overall problem of security easier or harder; on one hand, systems will become increasingly complex in construction and behavior and AI-based cyberattacks may be extremely effective, while on the other hand, the use of AI and machine learning techniques along with significant progress in low-level system reliability may render hardened systems much less vulnerable than today's," it states.
This potentially critical role that artificial intelligence will play in cyberwarfare makes it worthwhile investigating how to contain and limit the capabilities of such AI, according to the FLI.
"Very general and capable systems will pose distinctive security problems. In particular, if the problems of validity and control are not solved, it may be useful to create 'containers' for AI systems that could have undesirable behaviors and consequences in less controlled environments," it states.
Keeping control of AI
Ensuring humans can keep control of strong, autonomous AI is not straightforward.
For example, a system is likely to do its best to route around problems that prevent it from completing its desired task.
"This could become problematic, however, if we wish to repurpose the system, to deactivate it, or to significantly alter its decision-making process; such a system would rationally avoid these changes," the research points out.
The FLI recommend more research into corrigible systems, which do not exhibit this behaviour.
"It may be possible to design utility functions or decision processes so that a system will not try to avoid being shut down or repurposed," according to the research.
Another potential problem could stem from an AI negatively impacting its environment in the pursuit of its goals - leading the FLI to suggest more research into the setting of "domestic" goals that are limited in scope.
In addition, it recommends more work needs to be carried out into the likelihood and nature of an "intelligence explosion" among AI - where the capabilities of self-improving AI advance far beyond humans' ability to control them.
The research concludes on a hopeful note, speculating that given the right checks and balances that AI could transform societies for the better.
"Success in the quest for artificial intelligence has the potential to bring unprecedented benefits to humanity, and it is therefore worthwhile to research how to maximize these benefits while avoiding potential pitfalls."
Read more