Google releases source code of new on-device machine learning solutions
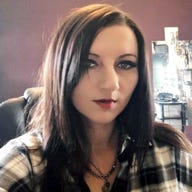
Google has opened up the source code of two machine learning (ML) on-device systems, MobileNetV3 and MobileNetEdgeTPU, to the open source community.
In a blog post, software and silicon engineers Andrew Howard and Suyog Gupta from Google Research said on Wednesday that both the source code and checkpoints for MobileNetV3, as well as the Pixel 4 Edge TPU-optimized counterpart MobileNetEdgeTPU, are now available.
Featured
On-device ML applications for responsive intelligence have been designed with power-limited devices in mind, including our smartphones, tablets, and Internet of Things (IoT) electronics.
See also: Google updates CallJoy phone agent with customizable AI features
Google says the demand for mobile intelligence has prompted research into algorithmically-efficient neural network models and hardware "capable of performing billions of math operations per second while consuming only a few milliwatts of power," such as in the case of the Google Pixel 4's Pixel Neural Core.
The latest MobileNet offerings include improvements to architectural design, speed, and accuracy, Google says. On mobile CPUs, users can expect MobileNetV3 to run at double the speed of MobileNetV2, bolstered through AutoML and NetAdapt, the latter of which has sliced away under-utilized activation channels.
CNET: Huawei ban: Full timeline as Trump's tech chief slams countries working with Chinese company
A new activation function called hard-swish (h-swish) has also been implemented to improve functionality on mobile devices and reduce the risk of bottlenecks. Overall latency has been decreased by 15 percent and object detection latency has been reduced by 25 percent in comparison to MobileNetV2.
The MobileNetEdgeTPU model -- similar to the Edge TPU in Coral products but tweaked for the camera features in Pixel 4 -- now also has increased accuracy in comparison to earlier versions, while reducing both runtime and power requirements.
Google did not set out to reduce the power demands of this model, but when compared to the basic MobileNetV3, MobileNetEdgeTPU consumes 50 percent less juice.
TechRepublic: IBM social engineer easily hacked two journalists' information
MobileNetV3 and MobileNetEdgeTPU code can now be accessed from the MobileNet GitHub repository.
Developers can also pick up a copy of open source implementation for MobileNetV3 and MobileNetEdgeTPU object detection from the Tensorflow Object Detection API page, and DeepLab is hosting the open source implementation for MobileNetV3 semantic segmentation.
Innovative artificial intelligence, machine learning projects to watch
Previous and related coverage
- Google forges cloud, data deal with major US health organization
- Google's plan to collect health data on millions of Americans faces federal inquiry
- Google Cloud rolls out platform for network visibility and management
Have a tip? Get in touch securely via WhatsApp | Signal at +447713 025 499, or over at Keybase: charlie0