Google's banana throwing robot is highly accurate
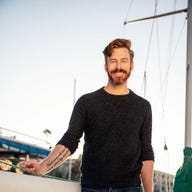
The ability to throw objects accurately offers all kinds of advantages. Tossing is an efficient way to move an object without engaging your whole body, for example. You can reach places you couldn't easily get to, as exemplified when tossing a set of keys from an apartment balcony to a friend on the street. And tossing objects is usually a quicker way to move them than carrying them.
"If you could get a robot arm to do that well," says Andy Zeng, a student researcher at Google, "that would make it much more versatile in the things it can do."
That's the premise behind a new tossing robot developed by Google researchers with collaboration from Columbia, MIT, and Princeton.
Tossing things, it turns out, is very difficult. Humans have a lifetime of practice but would be lucky to break 50 percent when throwing wadded up paper balls at a trashcan across a room. In that example, part of the problem is variance between the different paper balls, which make it tricky to dial in the physics behind the toss. If we were to exaggerate the problem by having a human reach into a box full of random objects -- a bowling pin followed by a pingpong ball, for example -- and toss them at a target, our success rate would be even lower.
Robots face a similar problem. From an engineering standpoint it's not particularly difficult to program a robot arm to toss a single object accurately. A basketball playing robot, for example, can shoot with near-perfect accuracy. But introducing variance in size, mass, and shape requires programming a robot that can develop a unique grasping strategy and account for the way an object will arc through the air on the fly.
The problem the TossingBot team wanted to solve was how to teach a robot to pick up random objects from a cluttered bin and place them in a box physically outside the robot's reach.
"TossingBot uses deep learning algorithms to associate the visual appearance of an object with how to grasp it and how to throw it," says Zeng. "Rather than having to manually engineer throwing strategies for each possible object, the robot can acquire these techniques through trial and error. Give it an object it has never seen before and it will learn by itself through trial and error how to throw them accurately."
Using overhead cameras to track where the object lands, the system learns from experience and gets better with each toss. When picking an object out of the bin, the robot uses a deep neural network to develop, in parallel, a grasping strategy and a tossing strategy.
The result is a robotic arm that can quickly pick and toss objects at a high rate of accuracy. The team is achieving a mean pick rate of 500 objects per hour.
Applications could include pick-and-place tasks in logistics centers and warehouses, for example. Tossing objects instead of physically placing them within the robot's maximum reach could have all kinds of advantages, including increasing throughput on lines and reducing the number of robot arms needed to distribute items into various bins.