Google's DeepMind turns to StarCraft II after conquering Go
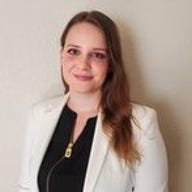
Google's DeepMind has announced that it will be making use of game development studio Blizzard's StarCraft II game as a testing platform for artificial intelligence (AI) and machine-learning research, opening the environment worldwide.
The announcement, made during Blizzard's BlizzCon conference at Anaheim on Sunday, has seen both companies build an open research environment to be used by anyone across the globe as of 2017, as well as by DeepMind itself.
"We've worked closely with the StarCraft II team to develop an API that supports something similar to previous bots written with a 'scripted' interface, allowing programmatic control of individual units and access to the full game state (with some new options as well)," DeepMind said.
"Ultimately, agents will play directly from pixels, so to get us there, we've developed a new image-based interface that outputs a simplified low-resolution RGB image data for map and minimap, and the option to break out features into separate 'layers', like terrain heightfield, unit type, unit health, etc."
The two are also working on creating "curriculum scenarios" -- a ladder of increasingly complex tasks so that AI researchers of any level can get their system running, benchmark algorithms, and advance. StarCraft II will also have editing tools to enable flexibility and control for the researchers taking part.
According to DeepMind, video games are one of the best ways to test and develop AI, with the company calling StarCraft II "the pinnacle of 1v1 competitive video games".
"DeepMind is on a scientific mission to push the boundaries of AI, developing programs that can learn to solve any complex problem without needing to be told how," DeepMind said.
"Games are the perfect environment in which to do this, allowing us to develop and test smarter, more flexible AI algorithms quickly and efficiently, and also providing instant feedback on how we're doing through scores."
DeepMind added that it has also used 2D games on Atari and complex games such as Go to test its AI system.
Featured
StarCraft II is closer to a real-world environment than any other game it has used for testing so far, DeepMind said, as it is played in real-time.
"The skills required for an agent to progress through the environment and play StarCraft well could ultimately transfer to real-world tasks," it claimed.
In playing StarCraft, the AI system will have to come up with real-time strategies for choosing one of the three distinct races at the beginning of the game; choosing when and how to farm minerals and gas; deciding when and which buildings and units to construct; and scouting unseen areas of the map and remembering that navigational information over the course of the game.
An AI engine would therefore have to make use of the skills of memory, mapping, long-term planning, and adapting to changes in plans using information that is continually being gathered, which translates to hierarchical planning and reinforcement learning.
Last month, researchers at DeepMind announced that they developed an AI that stores knowledge such as a map, allowing it to navigate such complicated systems as the London Underground.
DeepMind's differential neural computer (DNC) trains its memory through a process comparing its own answers with the correct answers, each time moving closer to producing correct answers itself.
"We wanted to test DNCs on problems that involved constructing data structures and using those data structures to answer questions. Graph data structures are very important for representing data items that can be arbitrarily connected to form paths and cycles," DeepMind researchers Alexander Graves and Greg Wayne explained in October.
"When we described the stations and lines of the London Underground, we could ask a DNC to answer questions like, 'Starting at Bond street, and taking the Central line in a direction one stop, the Circle line in a direction for four stops, and the Jubilee line in a direction for two stops, at what stop do you wind up?'. Or, the DNC could plan routes given questions like 'How do you get from Moorgate to Piccadilly Circus?'."
The DNC had an average accuracy of 98.9 percent after adding external memory.
DeepMind also recently announced developing a deep neural network that produces human-like text-to-speech systems.
The neural network closed the gap between machine-generated speech and human speech by approximately 50 percent, DeepMind said in September, for both US English and Mandarin Chinese speech.
DeepMind is also partnering with the United Kingdom's National Health Service to experiment with using machine learning to plan the use of radiotherapy for individual head and neck cancer patients, which could improve waiting times for procedures and free up more time for doctors nationwide.
"For these cancers, segmentation [radiotherapy planning] can take around four hours. And even though UCLH's specialist team at its dedicated head and neck cancer centre is a national leader in this process, there is still potential for innovation. We think machine learning could make a difference," DeepMind said in September.