IoT boom will change how data is analysed
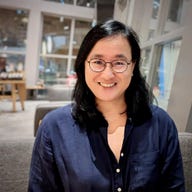
As more devices come online, each generating data, the way information is analysed and used to facilitate machine learning will have to change.
Data is key to improving the accuracy and predictions of machine learning and artificial intelligence (AI) systems--the more images of apple and oranges it is fed, the better it will be at distinguishing the two.
The "smartness" of a machine was data-driven, said Goh Eng Lim, vice president and CTO of high performance computing and AI, Hewlett-Packard Enterprise (HPE), who was speaking to media at the vendor's Reimagine Summit in Singapore.
However, he stressed, companies should not be focusing only on data retention as establishing volume alone was not adequate. Data also needed to be curated, labelled, and federated.
Goh noted that, too often, organisations operated data in silos, with the HR department generating data that did not integrate with data sitting with the sales team. In order to make better predictions, machine learning systems needed to be able to seamlessly pull data across the company.
They also needed to work on data that was properly labelled and curated to ensure decisions were made on accurate, quality data, he said.
Asked if the anticipated boom of IoT then would introduce further complications, he acknowledged the likelihood, adding that it could indeed worsen the situation.
The number of connected devices was estimated to have outpaced the global population last year at 8.4 billion, and would continue to climb to 20.4 billion by 2020, predicted Gartner.
For one, Goh noted, it would not be feasible to push all the data generated by every connected device back to the data centre to be analysed.
"The network can't keep up," he said. "Therefore, you'll need the IoT device to be smarter so it can make smart decisions at the edge, for instance, only sending back information that's needed back to the network."
The IoT device could ascertain if the data was of high quality and should be pushed back to the network to facilitate deep learning, or to process the learning at the edge and send back only the knowledge--rather than pure data.
The edge or IoT device would need to acquire more intelligence in order to carry out such decisions and tasks, Goh said.
HPE has been touting the importance of edge computing, peddling its range of HPE Edgeline systems, which it said would be necessary to support more compute and better manage data at the edge of the network.
Putting IoT compute at the edge also addressed latency as well as data sovereignty issues, said Mark Verbloot, HPE Aruba's Asia-Pacific Japan director of systems engineering, who was speaking at the summit.
It would enable data insights to be processed and accessed more quickly, Verbloot said.
In a 2016 ZDNet report, researchers at A*Star's Institute for Infocomm Research in Singapore said they had begun exploring technologies--specifically, distributed data analytics--that would enable data to be analysed more efficiently within the limited size and computational power of IoT devices.
Asked what remained blackholes in AI today, Goh noted that deep neural networks remained opaque. He explained that when these systems had made a prediction or decision that proved wrong, they were unable to determine what went wrong. Humans, too, were unable to provide further directions as it would be humanly impossible to trawl through all the data the neural networks had analysed to figure out what had gone wrong.
He also welcomed Singapore's move to set up an advisory council to assess the ethical and legal use of AI and data.
Noting that the same path was necessary when genome initiatives began, he said technology often moved ahead of policies and the latter needed to catch up. And robots already were advancing significantly, he added, pointing to the progress achieved by SoftBank's Boston Dynamics.
Goh further noted that machines depended on historical data to arrive at an answer, but what might be historically correct might not be socially accepted or defined to be "right". This underscored the need for humans to intervene and determine what was right or wrong.
"Humans grow up seeing the world [develop] and [learning] to assess what's right and wrong, We still need to be the supervisors of robots' decisions for, at least, many more years," he said, adding that this was why studies in humanities and social sciences were still essential.