Lies, damned lies and big data: How firms get analytics wrong – and how to get it right
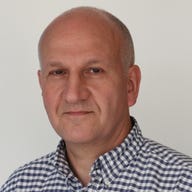
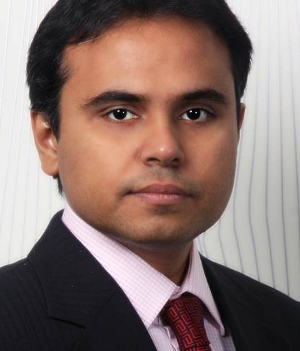
Petty company politics, bad data and inept analysis are standing between many organisations and any hope of a big-data utopia, where analytics routinely improve the business.
In companies where internal politicking is rife, people will deliberately bend analytics so the figures back up the course of action they support, warns Srikanth Velamakanni, founder and CEO of Fractal Analytics.
Even where there's no bias from vested interests, it's common to find errors caused by poor data or flawed analysis, he said.
"If you don't do analytics in the right manner, you can come up with some very wrong conclusions. I've seen so many examples — tons and tons of examples where companies make those mistakes," Velamakanni said.
He cited the case from a few years ago of trying to build a predictive churn model for a very large telecoms operator using inadequate data.
"We were trying to predict who was likely to cancel their subscriber line and what we could do using profitability, risk and lifetime value to retain them proactively," Velamakanni said.
Some of the models produced initially seemed extremely promising.
"They were highly predictive. They were so predictive that it was suspicious," he said.
At the time telecoms operators charged a small deposit for handsets and other equipment.
"One of the variables that was highly predictive was that customers who did not have a deposit were likely to leave. It was a very strong predictor of attrition," Velamakanni said.
"This seemed too good to be true. So we investigated and realised this company had a single field that said whether or not there's a deposit. What would happen is that if a customer left, the deposit would go out of view. So it was an after-the-fact variable.
"If you left, your deposit would go out of view but looking at the data you're not seeing that. You thought if they don't have a deposit, they are likely to leave. It was just a question of what was cause and what was effect, and in data you can't tell unless you get down to the detail."
Along with failures to examine the data closely, problems with the data itself rank high among the factors that can derail any big-data initiative.
"You're really handling large amounts of data with lots of messiness in it. There can be lots of missing values and all kinds of issues with what appears to be conflicting data which, when you get into it, you realise there's a lot of mess," Velamakanni said.
However, the one big danger he feels exists with analytics in general is the old adage about lies, damned lies and statistics.
"You can use analytics to prove a certain point and yet it could be a very faulty way of coming up with the analysis. This happens often and especially in very political organisations," he said.
Some clients are aware of the issue, and one told Velamakanni that he didn't want to 'democratise' analytics inside the company because it would be used by staff to fight political battles and justify conclusions that they thought were right.
Velamakanni believes the hurdles of internal bias and flawed interpretation can be overcome through the use of strong, standardised processes across an organisation and plenty of automation to clean out data errors.
"In some sense an audit trail of sorts is required so that errors can be detected and minimised. This is the dirty secret of the analytics world — that there are so many errors," he said.
"Many companies that create an analytics team and just start doing stuff, they make so many errors they don't even realise it, and that's why it's critical to create a strong process and make it error-free to deliver the right results."
Velamakanni rejects the idea that analytics should be kept in small specialised teams.
"The overall adoption of analytics is more critical than this challenge of people interpreting analytics in their own manner. In the beginning it will happen — there will be some instances of this," he said.
"But eventually the only way that adoption of analytics will grow and companies will get smarter through the use of analytics is if it is democratised."
More on big data and analytics
- Big data, 4G, and life-saving sensors: GM's vision of the smart car future
- Splunk, Ford project highlights big data, automobile mashup
- Kinesis: Amazon Web Services's answer to big data?
- IBM bets on big data visualization
- Hadoop and Big Data, "Stratafied"
- Splunk's big data Hunk gives Hadoop muscle to non-techies