MIT reveals AI platform which detects 85 percent of cyberattacks
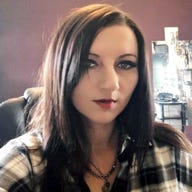
Today's cybersecurity professionals face daunting tasks: protecting enterprise networks from threats as best they can, damage limitation when data breaches occur, cyberforensics and documenting the evolution and spread of digital attacks and malware across the world.
It can be a challenge in an industry where many companies find themselves short-staffed and unable to find enough trained staff to maintain their ground against state-sponsored hackers which are tasked with stealing financial data, sensitive corporate intellectual property or spying upon unsuspecting victims.
Protecting infrastructure and people often is down to two sectors: man or machine. In today's current climate, there is a vast array of digital tools available to security professionals to streamline their tasks, but the bulk of the burden falls on human shoulders.
Could a new artificial intelligence platform change this? MIT researchers believe so.
On Monday, MIT's Computer Science and Artificial Intelligence Laboratory (CSAIL) said that while many "analyst-driven solutions" rely on rules created by human experts and therefore may miss attacks which do not match established patterns, a new artificial intelligence platform changes the rules of the game.
The platform, AI Squared (AI2), is able to detect 85 percent of attacks -- roughly three times better than current benchmarks -- and also reduces the number of false positives by a factor of five, according to MIT.
The latter is important as when anomaly detection triggers false positives, this can lead to lessened trust in protective systems and also wastes the time of IT experts which need to investigate the matter.
AI2 was tested using 3.6 billion log lines generated by over 20 million users in a period of three months. The AI trawled through this information and used machine learning to cluster data together to find suspicious activity. Anything which flagged up as unusual was then presented to a human operator and feedback was issued.
"You can think about the system as a virtual analyst," says CSAIL research scientist Kalyan Veeramachaneni. "It continuously generates new models that it can refine in as little as a few hours, meaning it can improve its detection rates significantly and rapidly."
AI2 is able to scan billions of log lines per day, assigning each piece of data as "normal" or "abnormal." The more attacks that come in and the more feedback which is given by human operators, the better, as AI2 learns what to look out for.
According to Veeramachaneni, this "cascading" effect can only improve the accuracy of future attack predictions.
MIT says the AI uses three different learning methods in order to show the top events at the end of each day for operators to label. The artificial intelligence platform then builds a model which is refined with input in what the team calls a "continuous active learning system."
The research institute says that on the first day of training, the AI will pick out the 200 most abnormal events for the operator to view. As the system learns, it identifies which events are actual attacks, and so within a matter of days analysts may only be viewing 30 or 40 events on a daily basis.
"This paper brings together the strengths of analyst intuition and machine learning, and ultimately drives down both false positives and false negatives," says Nitesh Chawla, the Frank M. Freimann Professor of Computer Science at the University of Notre Dame.
"This research has the potential to become a line of defense against attacks such as fraud, service abuse and account takeover, which are major challenges faced by consumer-facing systems."
Read on: Top picks