Snowflake and Databricks aim for dynamic duo
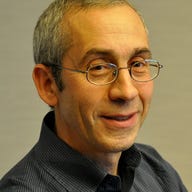
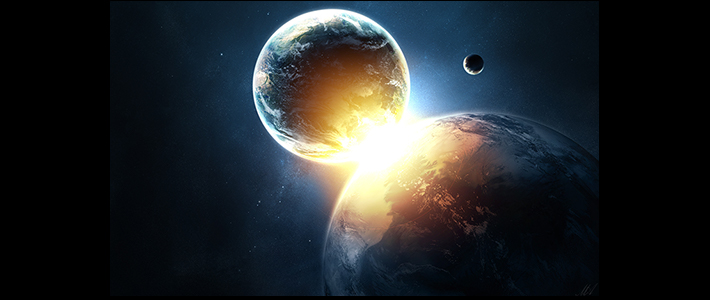
Snowflake and Databricks have more than their long association with the Amazon Web Services cloud in common: they have dozens of joint customers. It's the classic case of where different groups have their own tools: data scientists and data engineers who model and perform data engineering in Databricks, and business analysts who do query and reporting in Snowflake. Being in the AWS cloud means that they also share common storage of data in S3.
But for Snowflake and Databricks users, until now it's been a case of so near and so far. The services may have (hopefully) been in the same availability zone and stored in the same S3 instances. But until now, you had to work with generic connectors to get the two to tango.
Now both Snowflake and Databricks are formalizing the ad hoc relations that their joint clients have been developing.
This week, both announced that Databricks would include a new connector developed by Snowflake in its runtime. The connectors are, in effect, APIs for specific views of data residing in S3. The connector will be bi-directional: you can ingest Snowflake data into a Databricks Spark DataFrame, where it can be modeled, with the results viewed back in Snowflake. Alternatively, you could also use Databricks to perform the heavy lift of data engineering from non-Snowflake data sources, simply for performing ETL, or for enhancing the Snowflake data sets with additional external data.
For Databricks, this is the latest in a new series of connectors designed to expand the audience for its Spark-based analytic service. It follows up on recent connectors to R Studio, for meeting R programmers on their native ground, and with Alteryx, also for data engineering and analytics.
Snowflake is a strategic choice for Databricks as, unlike Amazon, there is no overlap between the product portfolios. There is also more of an impedance match with Snowflake, compared to Redshift as data warehousing target as both Databricks and Snowflake share S3 storage (although with Redshift Spectrum, that distinction gets a bit blurred). As such, data movement can be minimized for customers who consciously co-locate their use of Databricks and Snowflake in the same AWS availability zone and S3 storage instance.
Given the overlap, which both estimated with at least 40 - 50 customers, Snowflake and Databricks will follow up support of the connector with joint go to marketing and sales.