The dirtiest little secret about big data: Jobs
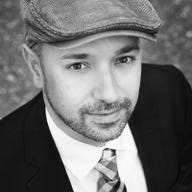

We like to think of big data as one of the most important advancements in the tech industry in recent years.
While the term itself has been abused for marketing purposes, the real concept behind big data--combining structured data (traditional business data) with unstructured data (new publicly available data sources)--produces new kinds of insights that were never before possible.
That's what makes the magic happen. And, we're seeing traditional businesses like Ford Motor Company and Microsoft using big data to help transform themselves--not to mention high flying upstarts like Facebook, Amazon, and Google that have become the next giants of international business by building their companies around data.
However, harnessing big data is anything but easy. Dealing with unstructured data requires mastering new types of database technologies such as NoSQL, Cassandra, and/or Hadoop. But, the even bigger problem isn't technological.
The No. 1 challenge that IT and business leaders face when it comes to big data is something that happens before it even gets to the fancy new databases or analytics software. It's more about business processes and people and jobs.
To illustrate it, let's look at a quick little story that will be familiar to most of you.
Earlier this year in a conference room, there was a sales representative from an analytics company giving what he clearly thought was a heckuva great presentation. His slide deck had charts and images and plenty of clever slide transitions. He looked confident that he had nailed it and was smiling--probably anticipating an easy sale--when he asked if he could clarify anything or answer any questions.
Slowly, a CIO spoke up and said, "That's great, but we already have several solutions that do the exact same thing. What I want to know is how easy is it for me to get my data into your system, because that's where all these other solutions fall down."
The smile suddenly wiped clean from the salesman's face, he stammered through a few platitudes and rattled off the number of data connectors his software had and how his team of technologists had an infinite number of data migrations under their belt, as the CIO listened, stony-faced. She'd seen this show before--and yet her company was still sitting on piles of valuable data that it couldn't organize into enough useful insights to change its business.
So, from that CIO's perspective, one of the key promises of the digital age--that big data will make us smarter, faster, and more efficient--remains an empty promise.
SEE: Big data's biggest problem: It's too hard to get the data in
Meanwhile, the companies that have figured out how to use big data well and turn it into a competitive advantage have overcome that one big obstacle.
Most of the companies we talk to tell us that the biggest frustration they have with big data is the amount of time and resources it takes to do data preparation, cleansing, sorting, scrubbing, and deduplication before the data can be analyzed and put to use.
Unfortunately, a lot of that falls on data scientists--some of the hottest (and most well-paid) jobs in tech right now. According to an Xplenty study, a third of today's high paid data wonks spend 50% to 90% of their time cleaning data before they can even analyze it.
As a result, many companies have tried to put machine learning and artificial intelligence to use in doing some of the data sorting and data cleansing. According to a Narrative Science survey, 58% of the companies that have big data solutions deployed, have also implemented AI.
Still, a lot of these companies quickly run up against the limitations of AI. It's excellent for very narrow, specific purposes. But, it's not very good at making judgment calls or deciding on something that falls into a gray area. For example, if we assigned an algorithm to examine all of the new videos published on YouTube last month and tell us how many were published about data centers versus how many were published about cloud computing, it would do great with the obvious things. But, it would struggle to throw out videos about rain clouds and cumulonimbus clouds, for example. It would also struggle with which bucket to place things like private cloud and hybrid cloud--even if we wrote allowances for some of those things into the algorithm.
Because of that, many companies have discovered that humans do the work of data sorting much better than algorithms, and so they are putting people to work behind the scenes to help their big data projects succeed. Some of them are hiring workers on their data teams while others are using labor marketplaces like Amazon Mechanical Turk to hire data labelers. This is likely part of the reason why the Narrative Science survey reported that 80% of the companies that have deployed AI have found that it ultimately creates additional jobs.
SEE: Is 'data labeling' the new blue-collar job of the AI era? (TechRepublic)
Thus, the dirtiest little secret about big data is that it's powered by small armies of people on the backend doing the low-tech work of manually organizing and sorting the data.
This phenomenon is likely to expand in the years ahead as more organizations are forced to embrace big data to stay competitive. Will the algorithms eventually catch up and automate this kind of data sorting? That remains to be seen. But for the near feature, the AI isn't going to be smart enough to handle all of it any time soon.
ZDNet Monday Morning Opener
The Monday Morning Opener is our opening salvo for the week in tech. As a global site, this editorial publishes on Monday at 8:00am AEST in Sydney, Australia, which is 6:00pm Eastern Time on Sunday in the US. It is written by a member of ZDNet's global editorial board, which is comprised of our lead editors across Asia, Australia, Europe, and the US.
Previously on Monday Morning Opener:
- Windows 10, Android, iOS, and the billion user question
- With AWS on deck, cloud rivals eye margin improvements, platform plays
- Why Trump and Brexit are an indictment of the tech industry and its worldview
- The internet as battleground: Where do we go from here?
- Apple winning the enterprise security race, Samsung makes push
- Tech after Brexit: Where do we go from here?
- Apple Car: Two big factors open the door for a disruptor
- Hey Siri! At Apple WWDC 2016, Tim Cook needs to make big data, AI pivot
- Death or rebirth: What does the future of the PC really look like?
- 7 Chinese companies that will shape the future of the tech industry: My week in Beijing
- March of the Chinese smartphones gathers pace