Why plunging into AI is a bad idea
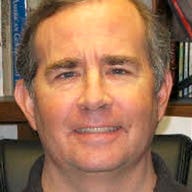
Featured
Given the hype surrounding artificial intelligence (AI), organizations might be tempted to plunge into AI initiatives without doing the necessary groundwork. The fact is, a lot can go wrong with AI, and the sooner organizations realize that and take precautions to avoid problems, the better.
AI in its many forms will increasingly permeate different aspects of business, said Anthony Scriffignano, senior vice president and chief data scientist at Dun & Bradstreet, a provider of analytics services to help companies customers improve their business performance.
"The challenges for leaders go well beyond the tools and techniques of AI, including knowledge retention, upgrading existing skills, and talent acquisition/retention," Scriffignano said. "Perhaps now more than ever, it is important for leaders to have a long view" of how AI will evolve.
Also: Can humans get a handle on AI?
Organizations and their data science teams need to avoid the rush to try every new AI method simply because of the attraction of something new, Scriffignano said. Instead, it's important to instill an understanding of preconditions, problem formulation, bias, and other analytical principles that are critical to any inference from data.
"Leading with a method or a tool is generally a bad idea," Scriffignano said. "One can't simply 'machine learn' out of a problem with new tools and technology." Instead, companies have to understand the types of problems they face that AI could address, and the appropriate approaches before rushing to apply a technology solution.
For example, consider an organization that's looking to understand social sentiment and how that sentiment is being influenced by marketing campaigns and sales activities.
"There are many tools available to do sentiment analysis, clustering, and other techniques," Scriffignano said. "It would be tempting to rush out and try one or more of these tools."
Must read
- Will AI need therapy in the future? (CNET)
- Top 5: Ways AI will change business (TechRepublic)
But companies need to first ask a few qualifying questions: Is there sufficient, readily available data? Is the data stable enough that conclusions will be valid? Is there a reason to think that the future can be extrapolated from prior data? Does the company have permissible use of the necessary data?
"Failing to answer such questions in advance could undermine the effort just as quickly -- if not more quickly -- than any technical issues," Scriffignano said.
Whenever lots of historical information exists, companies might have to deal with the challenge of "fake data" or bias.
"One obvious example of fake data would be false reviews--organizations that rate themselves, competitors who rate their competition harshly and falsely, and/or others who carry some unrelated grudge attempting to undermine the success of a company," Scriffignano said. "There are many popular sites where 'users' can provide reviews of products and services."
Also: How Facebook scales AI
Other examples of fake data might include falsified financial performance, false advertising claims, and information intended to influence the outcome of elections or to influence market behavior.
Companies need to be aware of the existence of these types of misleading data when delving into AI.
36 of the best movies about AI, ranked
Previous and related coverage:
What is AI? Everything you need to know
An executive guide to artificial intelligence, from machine learning and general AI to neural networks.
What is deep learning? Everything you need to know
The lowdown on deep learning: from how it relates to the wider field of machine learning through to how to get started with it.
What is machine learning? Everything you need to know
This guide explains what machine learning is, how it is related to artificial intelligence, how it works and why it matters.
What is cloud computing? Everything you need to know about
An introduction to cloud computing right from the basics up to IaaS and PaaS, hybrid, public, and private cloud.
Related stories:
- There is no one role for AI or data science: this is a team effort
- Startup Kindred brings sliver of hope for AI in robotics
- AI: The view from the Chief Data Science Office
- Salesforce intros Einstein Voice, an AI voice assistant for enterprises
- It's not the jobs AI is destroying that bother me, it's the ones that are growing