AI will transform product management
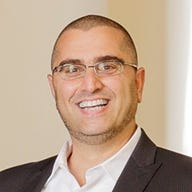
According to the World Economics Forum's The Future of Jobs 2018 report, machines will overtake humans in terms of performing more tasks at the workplace by 2025 -- but there could still be 58 million net new jobs created in the next five years. The report notes that the growing skills for 2022 will include analytical thinking, creativity, critical thinking, complex problem solving, and systems analysis.
Also: Can humans get a handle on AI?
The Future of Jobs Report 2018 also identified 10 emerging jobs in 2022, including data analysts and scientists, AI and machine learning specialists and general and operation managers as the top 3 jobs.
Also: How Facebook scales AI
AI and advancements in automation may result in 75 million job displacements, but at the same time period another 133 million new roles will emerge where people and machines will co-exist, creating a net new 58 million jobs by 2022. The 2018 jobs report also forecasts that 42 percent of all current tasks in the workplace will be performed by machines in 2022, as compared to 29 percent in 2018.
Also: Will AI need therapy in the future? CNET
I recently had the privilege of meeting Dr. Kai-Fu Lee, the Chairman and CEO of Sinovation Ventures and President of Sinovation Venture's Artificial Intelligence Institute, as he discussed his new book 'AI Superpowers - China, Silicon Valley and the New World Order' at my company's annual conference Dreamforce. Sinovation Ventures, managing US$2 billion dual currency investment fund, is a leading technology-savvy investment firm focusing on developing the next generation of Chinese high-tech companies. Dr. Lee's book is a must read for business leaders who want to better understand the impact of AI on the future of work. In his book, Dr. Lee identifies types of cognitive labor that is like to be displaced by AI and advance automation. The displacement likelihood of jobs are cataloged in various zones. The 'Danger Zone' which includes jobs like language translators, telemarketers, radiologists and tax specialists and insurance adjuster. The 'Safe Zone' includes social workers, CEOs and PR directors.
Also: AI means a lifetime of training CNET
I have written about my point of view regarding the massive impact of AI on the future of work. Fast forward to 2020, and I believe that sales, marketing, and customer service without AI is no longer effective or acceptable sales, marketing, or customer service. We are in the age of the connected stakeholder -- customers, business partners, employees, and communities. Every business, large and small, must transform to an AI-driven business. In the near term, companies and workers are less likely to be replaced by robots and more likely to be disrupted by companies and workers that are trained to use AI technologies to compete and win.
To better understand the impact of artificial intelligence (AI) on cognitive labor, I reached out to one of my AI domain expert colleagues who is responsible for delivering AI-powered products and services. Mayukh Bhaowal is Director of Product Management at Salesforce Einstein, working on automated machine learning. I was curious how he felt about the impact of AI on the future of product management function.
Also: Brewers are using AI to predict how your next beer will taste CNET
Here are Mayukh's thoughts on the future responsibilities of product management in the age of AI, and why product managers need to prepare differently as they bring to market AI-powered products and services:
On a massive stage in Cambridge, MA, in front of several hundred technology enthusiasts, ex-Stanford professor Andrew Ng scribbles away on a whiteboard. He is talking about how AI is changing the world, and how traditional job descriptions are breaking down. Ng gives an example of a chatbot. "Traditionally a Product Manager would provide product specifications through wire-frames. For a chatbot, wire-frames are completely useless to the engineers. They would like to know the logic inside," says Ng, who founded Coursera, an online education company, and who is now the CEO of AI consultancy Landing AI.
Jeremy Karnowski, Director of AI Products at San Francisco-based Insight Data Science, runs a 7-week intensive fellowship program which helps professionals with diverse backgrounds change careers and enter the field of software and technology. For example, Leah McGuire is a computational neuroscience who, after completing her Ph.D., joined Insight and landed a job at LinkedIn. Recently, Insight Data Science introduced a Data Product Management program. "It is for the more traditional product managers (PMs) to acquire the skills needed to be PMs in data-focused roles, like building products that leverage artificial intelligence," says Karnowski.
Marco Casalaina, VP of Product at Salesforce Einstein, is working with a team on creating a course in Trailhead (Salesforce's online learning platform) to augment the skill sets of traditional PMs and to prepare them for the fourth industrial revolution of AI and machine learning. "We need to develop a smell test for our PMs, to give them the ability to quickly determine the feasibility of applying machine learning to solve a business problem," says Casalaina.
Mayukh Bhaowal, Director of Product Management, Salesforce Einstein
Engineering as a discipline has existed for thousands of years. Its roots can be traced back to the engineering of the pyramids of Egypt, to the building of military engines in the 1300s, to the inception of mechanical engineering with the rise of the steam engine in the 1800s. This makes it a mature and well-established discipline.
Product management is relatively new, less than 100 years old and thus still evolving. It was originally meant to be around managing brands (hence known as Brand Men) as it evolved into managing production systems in manufacturing. The birth of software technology heavily influenced its course and then came along agile methodologies. Today, as Artificial Intelligence eats software, it is quietly transforming the product management role once again.
Also: 61% of businesses have already implemented AI TechRepublic
There is a shift. The AI product managers are rising.
Imagine building a new recommendation system. Given a product bought by a user, this system should recommend similar products. Traditionally, a PM would work with a user experience designer and come up with the user interaction and experience (UI/UX) specification around the layout, when and where to display the recommendations, what is the behavior on interacting with them, etc. This can be extended to other recommendation engine use cases, like recommending friends in a social network, connections in a professional network, songs in a music app, or job candidates in a recruiting system. However a modern recommendation system uses AI, and these kinds of product specifications are of no value to a machine learning engineer or a data scientist -- they need data. The systems they build are not coded; they're taught.
Let's say this recommendation system was destined to recommend job postings for job seekers, and vice versa. Here an AI product manager needs to provide particulars of the data that customers will have. What information do customers have about the jobs and the candidates? The PM needs to specify how the system functions in a "cold start," when there's a brand new customer who has not yet amassed enough data for the system to learn. And how do you evaluate that the recommendations are indeed good? This is a whole new breed of product specifications which did not exist until the recent dawn of AI.
In addition to working with their traditional cross-functional stakeholders (design, marketing, sales, engineering, dev ops), AI product managers now need to include data scientists and data engineers in the circle. Here are the top areas on which an AI product manager needs to focus beyond those of a traditional PM:
Problem Mapping
It is sometimes the case lately that in the rush to AI, PMs become mesmerized by the bevy of new technologies and neglect to map them to concrete user pain points. It is essential for the AI product manager to articulate the value proposition of an AI-powered feature. This means that an AI product manager should resort to AI methods of solving a business problem only after determining through careful evaluation that traditional methods such as rules engines will not adequately address the user's pain point.
In the customer service domain, for instance, the business problem might be accelerating the resolution of customer service tickets. One common problem that causes long resolution times in customer service is mis-categorized tickets: the more a ticket bounces between departments, the longer it takes to solve.
An AI product manager should do a careful analysis of this problem. Could it be solved by other means that are faster and easier to build than AI? In this particular example, businesses have long used rules engines and workflow systems to attempt to categorize cases correctly on the first try, and yet this problem persists. The rules in these engines frequently fall out of date, and even when they're current, they often require rigid inputs that don't match the range of human language that is often found in service tickets. Upon doing this analysis, an AI product manager might uncover an opportunity to use artificial intelligence to build a system to categorize these tickets by learning from prior successfully resolved tickets. This is a good example of an AI product manager mapping the high-level business problem to an AI solution.
Also: Top 5: Ways AI will change business TechRepublic
Data Literacy
One of the most significant aspects in the evolution of the AI product manager is the need for data literacy. An AI product manager needs to understand the right questions to ask about their customers' data if they are to succeed with an AI initiative. Is the relevant data readily available among customers? Is there enough data, and is it the kind of data from which AI can learn? Is the data clean or noisy? Does the use case require a real-time solution or would a batch solution suffice? Related to it, are there enough of prior examples or precedents which can be used to teach a machine learning system. That is typically known as a supervised problem. If there are no previous examples (also known as outcomes), the machine would have to learn without any supervision of precedents. This is known as an unsupervised problem.
For instance, a general-purpose image prediction software will perform poorly if it needs to detect tumors from medical images, but it would work well for classifying cats versus dogs. This is because it was never trained on (and hence did not learn) historical data of specific types of tumors and their outcomes. Often, there is not even enough such historical data to learn from.
This brand new dimension of data literacy is entirely absent in traditional PM roles.
Acceptance Criteria In The World Of AI
Featured
Once an AI-powered feature is built, AI product managers need to define the criteria to determine whether it is ready to serve production customers. In traditional product management, this criteria might include feature completeness, the number of open bugs, and fit and finish; but in AI product management, the key metric is AI accuracy. Data scientists have a broad set of machine learning metrics like lift, precision, and recall. Depending on the business objective, an AI product manager needs to make a judgment as to which machine learning metrics need to be optimized, and at what point the AI is performing sufficiently well to solve the customer's problem. This will then be mapped to the top line business metric such as case resolution time, or sales lead conversion lift.
For example, in the sales domain, sales executives are interested in increasing the number of incoming leads converting into sales. AI-based lead scoring can provide a prediction for every incoming lead as to whether it is likely to convert into a sale or not. This can help the salespeople focus their time on the right leads. The AI product manager thus needs to define a threshold of AI accuracy such that its use will reliably lift the conversion rate of customers' sales leads.
Also: 10 ways AI will impact the enterprise in 2018 TechRepublic
Explain-ability, Ethics, and Bias
"Why did this AI just make the decision that it did?" is a common question among users consuming AI. AI differs from traditional software in the sense that the outcomes are not based on a set of hand-coded rules; as such the decisions it makes can sometimes defy ready explanation. Additionally, the behavior of AI changes over time as it continues to learn.
Being thoughtful about explain-ability and providing mechanisms to make AI-powered features more transparent is the key to unlocking adoption of such features. An AI product manager needs to specify how his or her products will explain themselves to their users. Sometimes this involves a trade-off: some AI algorithms are more explainable than others. For problems in domains where there is high regulation, like healthcare, explain-ability beats accuracy. It is incumbent upon the AI product manager to strike the right balance for the use case and industry they are serving.
AI product managers also need to evaluate bias and consider the ethical implications of AI-powered applications. Is the data gathered representative and diverse enough? Is there data in the data-set which can introduce unwanted and unethical biases, like race or gender? This is hardly a simple technical decision. Gender, for example, can be a crucial signal for AI in medical diagnosis applications, but it is probably irrelevant in predicting which job applicant is more likely to fit a job description. It falls to the AI product manager to make decisions on which data is ethically fit for a given application.
Scaling From Research To Production
The attributes of a production AI application delivered at scale are very different than "data science projects" still in the research stage. Engineers who are taking AI applications to production need specifications around where the data is expected to be. Will it be online in a cloud environment? Or distributed across many mobile devices? They need to understand how quickly the AI needs to emit its output -- real-time AI output can be resource-intensive for both the engineers and the machines. There are numerous other considerations around how often and when machine learning models retrain, what metrics need to be computed to understand the system's continuing performance, what feature engineering is required, and much more. Cutting-edge methods of solving many common AI problems abound in academic publications, but very few of them scale up to real world applications. An AI PM needs to build that spidey sense around which problems to go after with the foresight, which of those will actually make into production.
Also: It's not the jobs AI is destroying that bother me, it's the ones that are growing
If you are a PM reading this and have experience building web and mobile applications but have not wet your feet in AI and ML (machine learning) products, you might be wondering -- "I had never had to deal with any of these!" That is precisely the point.
Though AI recently caught a lot of tailwinds, AI was prevalent even two decades back in the domain of search and Ad targeting. Some of you who worked on those early products leveraging AI and ML might be smiling now. As AI and ML eat software, more and more PMs need to level up their skills to manage these products and provide requirements and specifications which will add value to the data engineering and data science teams. This will lead to actually solving customer pain points and not just building a cool technology feature.
This article was co-authored by Mayukh Bhaowal, director of product management at Salesforce Einstein, working on automated machine learning.
36 of the best movies about AI, ranked
Previous and related coverage:
What is AI? Everything you need to know
An executive guide to artificial intelligence, from machine learning and general AI to neural networks.
What is deep learning? Everything you need to know
The lowdown on deep learning: from how it relates to the wider field of machine learning through to how to get started with it.
What is machine learning? Everything you need to know
This guide explains what machine learning is, how it is related to artificial intelligence, how it works and why it matters.
What is cloud computing? Everything you need to know about
An introduction to cloud computing right from the basics up to IaaS and PaaS, hybrid, public, and private cloud.