Graph theory suggests COVID-19 might be a ‘small world’ after all
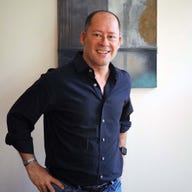
Is the spread of the respiratory infection known as COVID-19 happening in an "exponential" fashion?
That's been the general contention of the media, which, as a public service, have explained at some length the basics of fast-growing quantities, such as disease, to hammer home how something like a virus can double in cases in a matter of days.
However, the data on COVID-19 has a lot of puts and takes, and one of the factors not entirely considered is the graph of the infection. Graph theory has a lot to say about how phenomena can grow, such as the spread of infectious diseases. There are different graphs, or networks, of relations, and they can affect things such as the rate of propagation.
One particular recent work calls into question the notion of the exponential growth of the disease.
Scholars Anna Ziff and Robert Ziff, respectively of Duke University and the University of Michigan, earlier this month posted on the medrXiv pre-print server their curve-fitting exercise for COVID-19 confirmed cases and deaths, both in China and in the rest of the world, titled "Fractal kinetics of COVID-19 pandemic."
As the authors write, "in standard epidemiological analysis, one assumes that the number of cases in diseases like this one grows exponentially, based upon the idea of a fixed reproduction rate."
But that standard epidemiological view is not born out by the data. They found that while the numbers "display large growth, they do not, in fact, follow exponential behavior." Rather, the authors observed a period of initial exponential growth, followed by what's called a "power law," which is not the same thing.
Also: MIT's deep learning found an antibiotic for a germ nothing else could kill
"The behavior is clearly not linear as it would be for exponential growth," they write. "In contrast, we find that the data are very well fit by assuming a power-law behavior with an exponent somewhat greater than two."
The authors explain that growth by appeal to the notion of a "small world" network, where most people have only a small number of connections to other people, most of whom are neighbors within their community.
The small-world phenomenon was explored and revised substantially in the past two decades by graph theoretician Albert-László Barabási of Northeastern University, who runs The Center for Complex Network Research.
Anna L. Ziff and Robert M. Ziff argue the curve for deaths in China from Covid-19 isn't following the common "exponential" growth that mainstream epidemiology suggests; rather, it follows the "power law" of a "small world" network.
Barabási popularized the application of power laws to graphs with his 2014 book Linked. In that volume, Barabási pointed out that networks of infectious disease seem to follow a power law, whereby there is a steadily decreasing curve of connectedness between entities. For the vast majority of entities in a graph, such as, for example, the people who could potentially be infected, their connectedness dwindles at a rate that is less than exponential, the now-famous "long tail" that is familiar in internet content.
That doesn't mean disease can't spread fast, Barabási wrote. But he hypothesized that the small-world network must have "hubs," just like the major internet sites, to amplify the spread of signals.
The point is that a lot of real-world networks, such as infectious disease, are not random networks where there is an even chance of any one person coming into contact with another. They have structure, and the dominant structure of those networks is that the vast majority of people have a sparse number of connections to local neighbors. A random network would imply exponential growth, whereas the so-called scale-free networks that Barabási discovered do not. (If you want to dig into more about small-world networks, with excellent illustrations of how things propagate, especially disease, check out an excellent paper written by noted researcher Valdis Krebs, who is chief scientist with Orgnet, LLC.)
Also: Google DeepMind's effort on COVID-19 coronavirus rests on the shoulders of giants
Something like what Barabási discussed is identified in the Ziffs' paper. There is a tapering off that's been seen in the China data, they write, after an initial exponential curve. That suggests to them a "kind of small-world interaction network where individuals have many local neighbors and occasional long-range connections (such as caused by people traveling on trains, boats, and planes)."
The practical implication, if their model is correct, is that the speed of the disease is taking longer and longer to double in the number of deaths. Exponential growth is not maintained because "the number of susceptible individuals around an infected individual decays with time," as a result of the prospect that "Individuals already infected might face increased immunity and there are other individuals who might have had a mild infection that imparts immunity but without developing symptoms warranting testing."
"These various effects would inhibit the exponential growth of the virus."
The authors make a prediction, which is that starting from the 27th day of the outbreak, when there were 1,669 deaths from COVID-19, according to data compiled by the World Health Organization, the number of deaths would double to approximately 3,340 on day 37, which was Feb. 22, double again to 6,680 on day 50, March 10, and double again to 13,350 on day 67, which would be March 27.
It turns out, the doubling has been slower than they predicted, with actual deaths on day 37 coming in at just 2,359, and the death toll for March 10th at only 4,012. The total now, as of March 16, stands at 6,606, according to the WHO.
The Ziffs offer some thoughts on variance from their model. "Deviations above this power-law behavior might indicate that the pandemic is expanding from the current level of control," they write, "while deviations below might indicate that the disease is starting to fade."
Of course, there is a lot of uncertainty in the data on COVID-19 cases, which the authors acknowledge.
"This analysis is early in the outbreak of COVID-19 and cannot predict the length of the outbreak nor the final fatality," they write.
But the main point should be that the course of the disease is showing something other than the exponential growth that is routinely being tossed around. "This is a large number of fatalities," the Ziffs observe, "but not nearly as large as if the growth were exponential."
Keep in mind, then, as you think about infectious disease, that a simple rate of spread of something has to contend with the graph structure of a network, and that can have effects that are not obvious and that bear examination.