Machines that think like humans: Everything to know about AGI and AI Debate 3
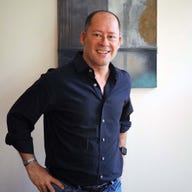
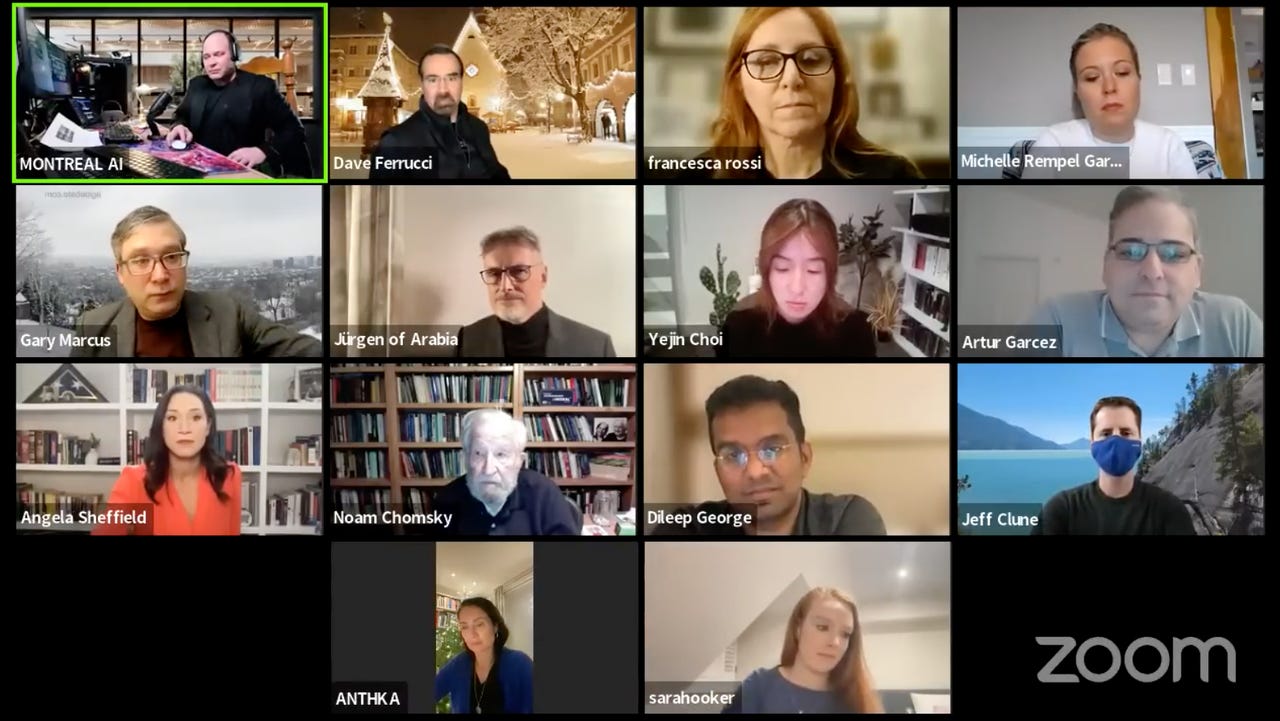
After a year's hiatus, the AI Debate hosted by Gary Marcus and Vincent Boucher returned with a gaggle of AI thinkers, this time including policy types and scholars outside of the discipline of AI such as Noam Chomsky.
After a one-year hiatus, the annual artificial intelligence debate organized by Montreal.ai and by NYU emeritus professor and AI gadfly Gary Marcus returned Friday evening, once again organized as a virtual-only event as in 2020.
The debate this year, AI Debate 3: The AGI Debate, as it's called, focused on the concept of artificial general intelligence, the notion of a machine capable of integrating a myriad of reasoning abilities approaching human levels.
While the previous debate featured a number of AI scholars, Friday's meet-up drew participation by 16 participants from a much wider gamut of professional backgrounds. In addition to numerous computer scientists and AI luminaries, the program included legendary linguist and activist Noam Chomsky, computational neuroscientist Konrad Kording, and Canadian parliament member Michelle Rempel Garner.
Also: AI's true goal may no longer be intelligence
Here's the full list: Erik Brynjolfsson, Yejin Choi, Noam Chomsky, Jeff Clune, David Ferrucci, Artur d'Avila Garcez, Michelle Rempel Garner, Dileep George, Ben Goertzel, Sara Hooker, Anja Kaspersen, Konrad Kording, Kai-Fu Lee, Francesca Rossi, Jürgen Schmidhuber and Angela Sheffield.
Marcus was once again joined by his co-host, Vincent Boucher of Montreal.ai.
The 3-hour program was organized around five panel topics:
Panel 1: Cognition and Neuroscience
Panel 2: Common Sense
Panel 3: Architecture
Panel 4: Ethics and morality
Panel 5: AI: Policy and Net Contribution
The debate ran longer than planned. The full 3.5 hours can be viewed on the YouTube page for the debate. The debate Web site is agidebate dot com.
In addition, you may want to follow the hashtag #agidebate.
NYU professor emeritus and AI gadfly Gary Marcus resumed his duties hosting the multi-scholar face-off.
Marcus started things off with a slide show of a "very brief history of AI," tongue firmly in cheek. Marcus said that contrary to enthusiasm in the decade following the landmark ImageNet success, the "promise" of machines doing various things had not paid off. He featured reference to his own New Yorker article throwing cold water on the matter. Nonetheless, Marcus said "ridiculing the skeptic became a pasttime" and critiques by him and others were pushed aside amid the enthusiasm for AI.
By the end of 2022, "the narrative began to change," said Marcus, citing negative headlines about Apple delaying its self-driving car, and negative headlines about the ChatGPT program from OpenAI. Marcus cited negative remarks by Meta's Yann LeCun in a conversation with ZDNET.
Marcus dedicated the evening to the late Drew McDermott of the MIT AI Lab, who passed away this year, and who wrote a critical essay in 1976 titled "Artificial intelligence meets natural stupidity."
Famed linguist and activist Noam Chomsky said today's impressive language models such as ChatGPT "are telling us nothing about language and thought, about cognition generally, or about what it is to be human." A different kind of AI is needed, he said, to answer the question of the Delphic Oracle, "What kind of creatures are we?"
Chomsky was the first speaker of the evening.
Chomsky said he had few things to say about AI's goals, to which he could not contribute. But he offered to speak on the topic of what cannot be achieved by current approaches to AI.
"The problem is quite general and important," said Chomsky. "The media are running major thought pieces about the miraculous achievements of GPT-3 and its descendants, most recently ChatGPT, and comparable ones in other domains, and their import concerning fundamental questions about human nature."
He asked, "Beyond utility, what do we learn from these approaches about cognition, thinking, particularly language, a core component of human cognition?"
Also: AI Debate 2: Night of a thousand AI scholars
Chomsky said, "Many flaws have been detected in large language models," and maybe they'll get better with "more data, more parameters." But, "There's a very simple and fundamental flaw that will never be remedied," he said. "By virtue of their design, the systems make no distinction between possible and impossible languages."
Chomsky continued,
The more the systems are improved, the deeper the failure becomes […] They are telling us nothing about language and thought, about cognition generally, or about what it is to be human. We understand this very well in other domains. No one would pay attention to a theory of elementary particles that didn't at least distinguish between possible and impossible ones […] Is there anything of value in, say, GPT-3 or fancier systems like it? It's pretty hard to find any […] One can ask, what's the point? Can there be a different kind of AI, the kind that was the goal of pioneers of the discipline, like Turing, Newell and Simon, and Minsky, who regarded AI as part of the emerging cognitive science, a kind of AI that what would contribute to understanding of thought, language, cognition and other domains, that would help answer the kinds of questions that have been prominent through millennia, with respect to the Delphic oracle, What kind of creatures are we?
Marcus followed Chomsky with his own presentation talking about four unsolved elements of cognition: abstraction, reasoning, compositionality, and factuality.
Also: ChatGPT is scary good at my job but can't replace me yet
Marcus then showed illustrative examples where programs such as GPT-3 fall down according to each element. For example, as regards "factuality," said Marcus, deep learning programs maintain no world model from which to draw conclusions.
Marcus offered a slide show on how AI falls down on four unsolved elements of cognition: abstraction, reasoning, compositionality, and factuality.
Konrad Kording, who is a Penn Integrated Knowledge Professor at the University of Pennsylvania, followed Marcus, in a pre-taped talk about brains. (Kording was traveling during the time of the event.)
Kording began by talking about the quest to bring notions of causality to computing. Humans, said Kording, are generally "bad detectives" but are good at "perturbing" things in order to observe consequences.
He described perturbing a microchip transistor, and following voltage traces to observe causality in practice. Humans are focused on rich models of the world, he said.
A slide from Konrad Kording's taped presentation.
Neuroscientist Dileep George, who focuses on AGI at Google's DeepMind unit, was next up. "I'm an engineer," he told the audience. George gave two examples of historic dates, the first airplane in 1903 and the first non-stop transatlantic flight in 1919, and challenged everyone to guess the year of the Hindenburg Disaster. The answer is 1937, which George said should be surprising to people because it followed so long after airplane progress.
Also: Devil's in the details in historic AI debate
The point, said George, was to distinguish between "scaling up" current AI programs, and understanding "fundamental differences between current models and human-like intelligence."
Marcus posed the first question of the speakers. He asked Chomsky about the concept of "innateness" in Chomsky's writing, the idea that something is "built in" in the human mind. Should AI pay more attention to innateness?
Said Chomsky, "any kind of growth and development from an initial state to some steady state involves three factors." One is the internal structure in the initial state, second is "whatever data are coming in," and third, "the general laws of nature," he said.
"Turns out innate structure plays an extraordinary role in every area that we find out about."
Things that are regarded as a paradigmatic example of learning, such as acquiring language, "as soon as you begin to take it apart, you find the data have almost no effect; the structure of the options for phonological possibilities have an enormous restrictive effect on what kinds of sounds will even be heard by the infant […] Concepts are very rich, almost no evidence is required to acquire them, couple of presentations […] my suspicion is that the more we look into particular things, the more we'll discover that as in the visual system and just about anything else we understand."
Neuroscientist Dileep George of DeepMind.
Marcus lauded the paper on a system called Cicero this year from Meta's AI scientists, because it ran against the thread of simply scaling up. He noted the model has separate systems for planning and language.
Artificial Intelligence
Dileep George chimed in that AI will need to make "some basic set of assumptions about the world," and "there is some magic about our structure of the world that enables us to make just some handful of assumptions, maybe less than half a dozen, and apply those lessons over and over again to build a model of the world."
Such assumptions are, in fact, inside of deep learning models, but at a level removed from direct observation, added George.
Marcus asked Chomsky, age 94, what motivates him. "What motivates me is the Delphic Oracle," said Chomsky. "Know thyself." Added Chomsky, there is virtually no genetic difference between human beings. Language, he said, has not changed since the emergence of humans, as evinced by any child in any culture being able to acquire language. "What kind of creatures are we?" is the question, said Chomsky. Chomsky proposed language will be the core of AI to figure out what makes humans so unique as a species.
Marcus moved on to the second question, about achieving pragmatic reasoning in computer systems. He brought out Yejin Choi, who is Brett Helsel Professor at the University of Washington, as the first speaker.
Choi predicted "increasingly amazing performances by AI in coming years."
Yejin Choi, Brett Helsel Professor at the University of Washington.
"And yet, these networks will continue making mistakes on adversarial and edge cases," Choi said. That is because "we simply do not know the depth" of the networks. Choi used the example of dark matter in physics, something science knows about but cannot measure. "The dark matter of language and intelligence might be common sense."
The dark matter here, said Choi, is the things that are easy for humans but hard for machines.
Next up was David Ferrucci, formerly of the IBM Watson project, and founder of a new company, Elemental Cognition.
Ferrucci said his interest always lay with programs with which he could converse as with a person. "I've always imagined we can't really get machines to fulfill this vision if they couldn't explain why they were producing the output they were producing."
Also: Mortal computing: An entirely new type of computer
Ferrucci gave examples of where GPT-3 fell down on common-sense reasoning tasks. Such systems, given enough data, "will start to reflect what we consider common sense." Poking around GPT-3, he said "is like exploring a new planet, it's sort of this remarkable thing," and yet "it's ultimately unsatisfying" because it's only about output, not about the reasons for that output.
His company, said Ferrucci, is pursuing a "hybrid" approach that uses language models to generate hypotheses as output, and then performing reasoning on top of that using "causal models." Such models "can be induced" from output, but they also require humans to interact with the machine. That would lead to "structured representations" on top of language models.
David Ferrucci, formerly of the IBM Watson project.
After Ferrucci, Dileep George returned to give some points on common sense, and "mental simulation." He discussed how humans will imagine a scenario -- simulating -- in order to answer common-sense reasoning questions. The simulation enables a person to answer many questions about a hypothetical question.
George hypothesized that the simulation comes from the sensorimotor system and is stored in the "perceptual+motor system."
Language, suggested George, "is something that controls the simulation." He proposed the idea of conversation as one person's attempt to "run the simulation" in someone else.
Marcus moved to Q&A. He picked up on Ferrucci's comment that "knowledge is projected into language."
"We now have this paradigm where we can get a lot of knowledge from large language models," said Marcus. That's one paradigm, he said. Marcus suggested "we need paradigm shifts," including one that would "integrate whatever knowledge you can draw from the language with all the rest." Language models, said Marcus, are "deprived" of many kinds of input.
Schmidhuber, who is scientific director of The Swiss AI Lab IDSIA (Istituto Dalle Molle di Studi sull'Intelligenza Artificiale), chimed in. Schmidhuber responded to Marcus that "this is an old debate," meaning, systems with a world model. "These are all concepts from the '80s and '90s" including the emphasis on "embodiment." Recent language models, said Schmidhuber are "limited, we know that."
Jürgen Schmidhuber, scientific director of The Swiss AI Lab IDSIA.
But, said Schmidhuber, there have been systems to address the shortcoming; things such as a separate network to predict consequences, to do mental simulations, etc.
"Much of what we are discussing today has, at least in principle, been solved many years ago," said Schmidhuber, through "general purpose neural networks." Such systems are "not yet as good as humans."
Schmidhuber said "old thinking" is coming back as computing power is getting cheaper every few years. "We can do with these old algorithms a lot of stuff that we couldn't do back then."
Marcus asked Schmidhuber and George about the old experiment of SHRDLU conducted by one of Minsky's students, Terry Winograd, including the "blocks world" of simple objects to be named and manipulated. Marcus said he has raised as a challenge, Could DeepMind's "Gato" neural network be able to do what blocks world failed to do? "And would it be a reasonable benchmark for AI to try to do what Winograd did in his dissertation in the 1960s?"
George replied that if it's about a system that can acquire abstractions from the world, and perform tasks, such as stacking things, such systems haven't been achieved.
Schmidhuber replied that computer systems already do well at manipulating in simulations, such as video games, but that in the real world it's a matter of choosing new experiments, "like a scientist," asking "the right questions."
"How do I invent my own programs such that I can efficiently learn through my experiments and then use that for my improved world model?" he proposed.
"Why is it not yet like the movies?" asked Schmidhuber.
"Because of issues like that, few training examples that have to be exploited in a wise way, we are getting there, through purely neuron methods, but we are not yet there. That's okay because the cost of compute is not yet where we want it to be."
Marcus added that there are the real world and the simulated world and the issue of trying to link them via language. He cited a natural language statement from blocks world. The level of conversation in blocks world, said Marcus, "I think is still hard even in simulation" for current AI to achieve.
Marcus then turned to Artur d'Avila Garcez, professor of computer science at City, University of London. d'Avila Garcez offered that the issue is one, again, of innateness. "We are seeing a lot recently of constrained deep learning, adding these abstractions." Such programs are "application specific."
D'Avila Garcez said it might be a matter of incorporating symbolic AI.
Choi commented that "in relation to innateness, I believe there's something to be learned from developmental psychology," regarding agency and objects and time and place. "There may be some fundamental rethinking we need to do to handle some concepts differently."
Said Choi, "I don't think the solution is in the past," and "just putting layers of parameters." Some formal approaches of the previous decades may not be compatible with the current approaches.
George chimed in that certain elements such as objects may "emerge" from lower level functioning. Said George, there was an assumption that "space is built in," but now, he believes it could be derived from time. "You can learn spatial representations purely form sensory motor sequences -- just purely time and actions."
Francesca Rossi, who is IBM fellow and AI Ethics Global Leader at the Thomas J. Watson Research Center, posed a question to Schmidhuber: "How come we see systems that still don't have capabilities that we would like to have? How do you explain that, Jurgen, that those defined techniques are still not making into current systems?"
Schmidhuber: "I am happy to answer that. I believe it's mostly a matter of computational cost at the moment."
Continued Schmidhuber,
A recurrent network can run any arbitrary algorithm, and one of the most beautiful aspects of that is that it can also learn a learning algorithm. The big question is which of these algorithms can it learn? We might need better algorithms. The option to improve the learning algorithm. The first systems of that kind appeared in 1992. I wrote the first paper in 1992. Back then we could do almost nothing. Today we can have millions and billions of weights. Recent work with my students showed that these old concepts with a few improvements here and there suddenly work really beautifully and you can learn new learning algorithms that are much better than back-propagation for example.
Marcus closed the section with the announcement about a new paper by himself and collaborators, called "Benchmarking Progress for Infant-level Physical Reasoning in AI," which he offered is "very relevant for the things we were just talking about." Marcus said the paper will be posted to the debate Web site.
Marcus then brought in Ben Goertzel, who wears multiple hats, as chief scientist at Hanson Robotics and head of the SingularityNET Foundation and the AGI Society, to kick off the third section, noting that Goertzel had helped to coin the term "AGI."
Ben Goertzel, chief scientist at Hanson Robotics.
Goertzel noted his current project is intent on building AGI and "embodied generally intelligent systems." He suggested systems that can "leap beyond their training" are "now quite feasible," though they may take a few years. He also prophesied the current deep learning programs won't make much progress to AGI. ChatGPT "doesn't know what the hell it's talking about," said Goertzel. But if combined with "a fact checker, perhaps you could make it spout less obvious bullshit, but you're still not going to make it capable of generalization like the human mind."
"General intelligence," said Goertzel will be more capable of imagination and "open-ended growth."
Also: Stack Overflow temporarily bans answers from ChatGPT
Marcus added "I completely agree with you about meta-learning," adding, "I love that idea of many roads to AGI."
Marcus then took a swipe at the myth, he said, that Meta's Yann LeCun and fellow Turing Award winners Geoffrey Hinton and Yoshua Bengio had pioneered deep learning. He introduced Schmidhuber, again, as having "not only written the definitive history of how deep learning actually developed, but he too has been making many major contributions to deep learning for over three decades."
"I'm truly most fascinated by this idea of meta-learning because it seems to encompass everything that we want to achieve," said Schmidhuber. The crux, he said, was "to have the most general type of system that can learn all of these things and, depending on the circumstances, and the environment, and on the objective function, it will invent learning algorithms that are properly suited for this type of problem and for this class of problems and so on." All of that is possible using "differentiable" approaches, said Schmidhuber.
Added Schmidhuber, "One thing that is really essential, I think, is this artificial scientist aspect of artificial intelligence, which is really about inventing automatically questions that you would like to have answers."
Schmidhuber summed up by saying all the tools exist, and, "It is a matter of putting all these puzzle pieces together."
Marcus offered that he "completely agrees" with Schmidhuber about the principle of meta-learning, if not the details, and then brought up Francesca Rossi.
Rossi titled her talk "Thinking fast and slow in AI," an allusion to Daniel Kahneman's book of the same title, and described a "multi-agent system" called "SOFAI," or, Slow and Fast AI, that uses various "solvers" to propose solutions to a problem, and a "metacognitive" module to arbitrate the proposed solutions.
Rossi went into detail describing the combination of "two broad modalities" called "sequential decisions in a constrained grid" and "classical and epistemic symbolic planning."
Rossi made an emphatic pitch for the human in the loop, stating that the combined architecture could extend the human way of making decisions "to non-humans," and then, have the machine "interact with human beings in a way that nudges the human to use his System One, or his or her System Two, or his or her metacognition."
"So, this is a way to push the human to use one of these three modalities according to the kind of information that is derived by the SOFAI architecture." Thus, thinking fast and slow would be a model for how to build a machine, and a model for how it interacts with people.
Francesca Rossi, IBM fellow and AI Ethics Global Leader at the Thomas J. Watson Research Center.
Following Rossi, Jeff Clune, associate professor of computer science at the University of British Columbia, was brought up to discuss "AI-generating algorithms: the fastest path to AGI."
Clune said that today's AI is using a "manual path to AI," by "identifying building blocks." That means all kinds of learning rules and objective functions, etc -- of which he had a whole slide. But that left the challenge of how to combine all the building blocks, "a Herculean challenge," said Clune.
In practice, hand-designed approaches give way to automatically generated approaches, he argued.
Clune proposed "three pillars" in which to push: "We need to meta-learn the architectures, we need to meta-learn the algorithms, and most important, we need to automatically generate effective learning environments and/or the data."
Clune observed AI improves by "standing on the shoulders" of various advances, such as GPT-3. He gave the example of the OpenAI project where videos of people playing games brought a "massive speed-up" to machines playing Minecraft.
Clune suggested adding a "fourth pillar," namely, "leveraging human data."
Clune predicted there is a 30% change of achieving AGI by 2030, as defined as "capable of doing more than 50% of economically valuable human work." Clune added that the path is "within the current paradigm," meaning, no new paradigm was needed.
Clune closed with the statement, "We are not ready" for AGI. "We need to start planning now."
Marcus said "I'll take your bet" about a 30% chance of AGI in 2030.
Jeff Clune, associate professor of computer science at the University of British Columbia.
Clune was followed by Sara Hooker, who heads up the non-profit research lab Cohere For AI, talking on the topic, "Why do some ideas succeed and others fail?"
"What are the ingredients we need for progress?" Hooker proposed as the issue.
It took three decades from the invention of back-prop and convolutions in the '60s, '70s, and '80s, to get to deep learning being recognized. Why? she asked.
The answer was the rise of GPUs, she said. "A happy coincidence."
"It's really the luck of ideas that succeeded being compatible with the available tooling," Hooker offered.
Sara Hooker, who heads up the non-profit research lab Cohere For AI.
The next breakthrough, said Hooker, will require new algorithms and new hardware and software. The AI world has "doubled down" on the current framework, she said, "leaning in to specialized accelerators," where "we've given up flexibility."
The discipline, said Hooker, has become locked into the hardware, and with it, bad "assumptions," such as that scaling of neural nets alone can succeed. That, she said, raises problems, such as the great expense of "memorizing the long tail" of phenomena.
Progress, said Hooker, will mean "reducing the cost of different hardware-software-algorithm combinations."
Marcus then brought up D'Avila Garcez again to give his slide presentation on "sustainable AI."
"The time is right to start talking about sustainable AI in relation to the U.N.'s sustainability goals," said D'Avila Garcez.
D'Avila Garcez said the limitations of deep learning have become "very clear" since the first AI debate, including, among others, fairness, energy efficiency, robustness, reasoning, and trust.
"These limitations are important because of the great success of deep learning," he said. D'Avila Garcez proposed neuro-symbolic AI as the path forward. Relevant work on neural-symbolic goes back to the 1990s, said D'Avila Garcez, and he cited background material at http://www.neural-symbolic.org.
D'Avila Garcez proposed several crucial tasks, including "measures of trust and accountability," and "fidelity of an explanation" and alignment with sustainable development goals, and not just "accuracy results."
Artur d'Avila Garcez, professor of computer science at City, University of London.
Curriculum learning will be especially important, said D'Avila Garcez. A "semantic framework" for neural-symbolic AI is a new paper coming out shortly, he noted.
Clune kicked off the Q&A, with the question, "Wouldn't you agree that ChatGPT has fewer of those flaws than GPT-3, and GPT-3 has fewer than GPT-2 and GPT-1? If so, what solved those flaws is the same playbook, just scaled up. So, why should we now conclude that we should stop and add more structure rather than embrace the current paradigm of scaling up?"
George offered the first response. "It's clear scaling will bring some results that are more impressive," he said. But, "I don't agree the flaws are fewer," said George. "Systems are improving, but that's a property of many algorithms. It's a question of the scaling efficiency."
Also: Meta's Data2vec 2.0: Second time around is faster
Choi replied, "ChatGPT is not larger than GPT-3 DaVinci, but better trained through a huge amount of human feedback, which comes down to human annotation." The program, said Choi, has produced rumors that "they [OpenAI] might have spent over $10 million for making such a human annotated data. So, it's maybe a case of more manual data." Choi rejected the notion ChatGPT is better.
Said Choi, the programs are exciting, but "I really don't think we know what are the breadth and depth of the corner cases, the edge cases we might have to deal with if we are to achieve AGI. I suspect it's a much bigger monster than we expect." The gap between human intelligence and the next version of GPT is "much bigger than we might suspect today."
Marcus offered his own riposte: "The risk here is premature closure, we pick an answer we think works but is not the right one."
The group then adjourned for 5 minutes.
After the break, Canadian Parliament member Michelle Rempel Garner was the first to present.
Marcus introduced Rempel Garner as one of the first people in government to raise concerns about ChatGPT.
Rempel Garner offered "a politician's take." The timeline of the arrival of AGI is "uncertain," she said, but as important is the lack of certainty of how government should approach the matter. "Efforts that have emerged are focused on relatively narrow punitive frameworks." She said that was problematic for being a "reactive" posture that couldn't match the speed of the development of the technology. "Present discourse on the role of government tends to be a naive, pessimistic approach."
Canadian Parliament member Michelle Rempel Garner.
Government, said Rempel Garner, holds important "levers" that could be helpful to stimulate the broad research outside of just private capital. But governments face problems in utilizing their levers. One is that social mores haven't always adapted to the new technology. And governments are always operating in a context where they assume humans as the sole forms of cognition. Government isn't set up to consider humanity sharing the planet with other forms of cognition.
Rempel Garner said, "My concern as legislator is our government is operating in a context where humans are the apex of mastery," but, "government should imagine a world where that might not be the case."
Rempel Garner offered "lived experience," in attempting to pass a bill in Parliament about Web3. She was surprised to find it became a "partisan exercise" and said, "This dynamic can not be allowed to repeat itself with AGI."
She continued, "Government must innovate and transcend current operating paradigms to meet the challenges and opportunities that the development of AGI presents; even with the time horizon of AGI emergence being uncertain, given the traditional rigidity of government, this needed to start yesterday."
Also: White House passes AI Bill of Rights
Choi followed Rempel Garner with a second presentation, to elaborate upon her position about how "AI safety," "Equity" and "Morality" would be seen in conjunction.
Choi cited bad examples of ChatGPT violating common sense and offering dangerous suggestions in response to questions.
Choi described the "Delphi" research prototype to build "commonsense moral models" on top of language models. She said Delphi would be able to speculate that some utterances could be dangerous suggestions.
Delphi could "align to social norms" through reinforcement learning, but not without some "prior knowledge," said Choi. The need to teach "human values" leads, said Choi, to a need for "value pluralism," the ability to have a "safe AI system" that would respect diverse cultural systems.
Marcus then brought up Anja Kaspersen from the Carnegie Council on AI Ethics.
Kaspersen shared six observations to promote discussion on the question of "Who gets to decide?" with respect to AGI, and "Where does AI stand with respect to power distribution?"
Her first theme was "Will the human condition be improved through digital technologies and AI, or will AI transform the human condition?"
"I see there is a real risk in the current goals of AI development that could distort what it means to be human," observed Kaspersen. "The current incentives are geared more toward the replacement side [i.e., replacing humans] rather than AI systems which are aimed at enhancing human dignity and well-being," she said.
"If we do not redirect all that … we risk becoming complicit and destroying the foundation of what it means to be human and the value we give to being human and feeding into autocracy," she said.
Second, "the power of narratives and the perils of what I call unchecked scientism."
Anja Kaspersen, senior fellow with the Carnegie Council on AI Ethics.
"When discussing technologies with a deep and profound impact on social and political systems, we need to be very mindful about providing a bottoms-up, reductionist lens, whereby scientism becomes something of an ethology."
There is a danger of "gaslighting of the human species," she said, with narratives that "emphasize flaws in individual human capabilities."
Kaspersen cited the example of scientist J. Robert Oppenheimer, who gave a speech in 1963: "He said, It is not possible to be a scientist unless you believe knowledge of the world and the power which this gives is a thing which is of intrinsic value for humanity." Oppenheimer's notion of consequences, she said, has increasingly moved from people in decision making to now the scientists themselves.
Third, AI is a "story about power," she said. The "current revolution" of data and algorithms is "redistributing power." She cited Marcus's point about the pitfalls of "decoupling."
"People who try to speak up are gaslighted and pushed back and bullied," she said.
Fourth, she said, is the "power of all social sciences," borrowing from sociologist Pierre Bourdieu, and the question of who in a society have "the biggest interest in the current arrangements" and in "keeping certain issues away from the public discourse."
Fifth, said Kaspersen, was the problem that ways of addressing AI issues become a "governance invisibility cloak," a way to "mask the problems and limitations of our scientific methods and systems in the name of addressing them."
Kaspersen's sixth and final point was that there is "some confusion as to what it means to embed ethics in this domain," meaning AI. The key point, said Kaspersen, is that computer science is no longer merely "theoretical," rather, "It has become a defining feature of life with deep and profound impact on what it means to be human."
Following Kaspersen, Clune and Rossi offered brief remarks in response.
Clune said he was responding to the question, "Should we program machines to have explicit values?"
"My answer is, no," he said. The question is "critical," he said, "because AGI is coming, it will be woven into and impact nearly every aspect of our lives."
Clune said, "The stakes couldn't be higher; we need to align AI's ethics and values with our own." But, values should not be hard-coded because, "No. 1, all signs point to [the idea that] the learned AI or AGI will vastly outperform anything that's hand-coded … and society will end up adopting the most powerful AI available." And reason No. 2, "we don't know how to program ethics," he said. "It's too complex," he said, adding, "See Asimov's work on the Three Laws, for example," referring to Isaac Asimov's Three Laws of Robotics.
Clune suggested the best way to come at ethics is using reinforcement learning combined with human feedback, including a "never-ending stream of examples, discussions, debates, edge cases."
Clune suggested the gravest danger is what happens when AI and AGI "are made by unscrupulous actors," especially as large language models become easier and easier to train.
Rossi posited that "the question is whether we should embed values in AI systems," stating, "My answer is yes."
Values cannot be gotten "just by looking at data or learning from data," said Rossi, so that "it needs human data, it needs rules, it needs a neural-symbolic approach for embedding these values and defining them." While it may not be possible to hard-code values, said Rossi, "at some point they need to explicitly be seen from outside."
Following Rossi, Ferrucci offered a brief observation on two points. One, "Transparency is critical," he said, "AI systems should be able to explain why they're making the decisions they're making." But, he said, transparency doesn't obviate the need for a value system that is "designed somewhere and agreed upon."
Ferrucci said, "You don't want to be in a situation where a horrible decision is made, and it's OK just that you can explain it in the end; you want those decisions to conform to something that collectively makes sense, and that has to be established ahead of time."
Choi followed Ferrucci, observing that humans often disagree on what's moral. "Humans are able to know when people disagree," she noted. "I think AI should be able to do exactly that," she said.
Erik Brynjolfsson of the Stanford Institute for Human-Centered AI.
For the final segment, Erik Brynjolfsson, who is the Jerry Yang and Akiko Yamazaki Professor and Senior Fellow at the Stanford Institute for Human-Centered AI (HAI) and Director of the Stanford Digital Economy Lab, began by noting that "the promise of human-like AI is to increase productivity, to enjoy more leisure, and perhaps most profoundly, to improve our understanding of our own minds."
Some of Alan Turing's "Imitation Game" is becoming more realistic now, said Brynjolfsson, but there is another strain besides imitating humans, and that is AI that does things that augment or complement humans.
Also: The new Turing test: Are you human?
"My research at MIT finds that augmenting or complementing humans has far greater economic benefit than AI that merely substitutes or imitates human labor," said Brynjolfsson.
Unfortunately, he said, three types of stakeholders -- technologists, industrialists, and policymakers -- have incentives that "are very misaligned and favor substituting human labor rather than augmenting it."
And, "If society continues to pursue many of the kinds of AI that imitates human capabilities," said Brynjolfsson, "we'll end up in an economic trap." That trap, which he calls the Turing Trap, would end up creating rather perverse benefits.
"Imagine if Daedalus had developed robots" in the Greek myth, he said. "They succeed in automating tasks at that time, 3,000 years ago, and his robots succeeded in automating each of the tasks that ancient Greeks were doing at that time" such as pottery and weaving. "The good news is, human labor would no longer be needed, people would live lives of leisure, but you can also see that would not be a world with particularly high living standards" by being stuck with the artifacts and production of the time.
"To raise the quality of life substantially," posited Brynjolfsson, "we can't build machines that merely substitute for human labor and imitate it; we must expand our capabilities and do new things."
In addition, said Brynjolfsson, automation increases the concentration of economic power, by, for example, driving down wages.
Today's technology doesn't augment human ability, unlike the technologies in the preceding 200 years that had enhanced human ability, he argued.
"We need to get serious about technologies that complement humans," he said, to both create a bigger economic pie but also one that distributes wealth more evenly. The systemic issue, he argued, is the perverse incentives of the three stakeholders, technologists, industrialists, and policymakers.
Brynjolfsson was followed by Kai-Fu Lee, former Google and Microsoft exec and now head of venture capital firm Sinovation Ventures. Lee, citing his new book, AI 2041, proposed to "debate myself."
Kai-Fu Lee, former Google and Microsoft exec and now head of venture capital firm Sinovation Ventures.
In the book, he offers a largely positive view of the "amazing powers" of AI, and in particular, "AIGC," AI-generated content, including the "phenomenal display of capabilities that certainly exhibit an unbelievably capable, intelligent behavior," especially in commercial realms, such as changing the search engine paradigm and even changing how TikTok functions for advertising.
"But today, I want to mostly talk about the potential dangers that this will create," he said.
In past, said Lee, "I could either think of algorithms or at least imagine technological solutions" to the "externalities" created by AI programs. "But now I am stuck because I can't easily imagine a simple solution for the AI-generated misinformation as specifically targeted misinformation that's powerful commercially," said Lee.
What if, said Lee, Amazon Google or Facebook can "target each individual and mislead […] and give answers that could potentially be very good for the commercial company because there is a fundamental misalignment of interests, because large companies want us to look at products, look at content and click and watch and become addicted [so that] the next generation of products […] be susceptible to simply the power of capitalism and greed that startup companies and VCs will fund, activities that will generate tremendous wealth, disrupt industries with technologies that are very hard to control."
That, he said, will make "the large giants," companies that control AI's "foundation models" even more powerful.
"And the second big, big danger is that this will provide a set of technologies that will allow the non-state actors and the people who want to use AI for evil easier than ever." Lee suggested the possibility of a Cambridge Analytica-style scandal much worse than Cambridge Analytica had been because it will be powered by more sophisticated AIGC. Non-state actors, he suggested, might "lead people to thoughts" that could disrupt elections, and other terrible things --- "the beginning of what I would call "Cambridge Analytica on steroids."
Lee urged his peers to consider how to avert that "largest danger."
He said, "We really need to have a call of action for people to work on technologies that will harness and help this incredible technology breakthrough to be more positive and more controlled and more contained in its potential commercial evil uses."
Marcus, after heartily agreeing with Lee, introduced the final speaker, Angela Sheffield, who is the senior director of artificial intelligence at D.C.-area digital consulting firm Raft.
Angela Sheffield, senior director of artificial intelligence at D.C.-area digital consulting firm Raft.
Sheffield, citing her frequent conversations with "senior decision makers in the U.S. government and international governments," said that "there is a real sense of urgency among our senior decision makers and policymakers about how to regulate AI."
"We might know that AGI isn't quite next," said Sheffield, but it doesn't help policymakers to say that because "they need something "right now that helps them legislate and helps them also to engage with senior decision makers in other countries."
"More than just frameworks" are needed, she said. "I mean, applying this to a meaningful real-world problem, setting the parameters of the problem, being specific […] something to throw darts at. The time is now to move faster because of the motivation we just heard from Kai-Fu."
She posed the question, "If not us, then who?"
Sheffield noted her own work on AI in national security, particularly with respect to nuclear proliferation. "We found some opportunities where we could match AI algorithms with new data sources to enhance our ability to augment the way that we approach finding bad guys developing nuclear weapons." There is a hope, said Sheffield, of using AI to "augment the way that we do command and control," meaning, "how a group of people takes in information, makes sense of it, decide what to do it, and execute that."
For the concluding segment, Marcus declared a "lightning round," the chance for each participant to answer, in 30 seconds or less, "If you could give students one piece of advice on, for example, What AI question most needs researching, or how to prepare for a world in which AI is increasingly central, what would that advice be?"
Brynjolfsson said there has been "punctuated equilibrium" improvement in AI, but there has not been such improvement in "our economic institutions, our skills, our organizations," and so the key is "how can we reinvent our institutions."
Ferrucci offered: "Your brain, your job, and your most fundamental beliefs will be challenged by AI like nothing ever before. Make sure you understand it, how it works, and where and how it is being used."
Choi: "We've got to deal with aligning AI with human values, especially with an emphasis on pluralism; I think that's one of the really critical challenges we are facing and more broadly addressing challenges such as robustness, and generalization and explainability and so forth."
Kaspersen: "Break free of silos; it is a type of convergence of technologies that is simply unprecedented. Ask really good questions to navigate the ethical considerations and uncertainties."
George: "Pick whether you want to be on the scaling one or on the fundamental research one, because they have different trajectories."
D'Avila Garcez: "We need an AI that understands humans and humans that understand AI; this will have a bearing on democracy, on accountability, and ultimately education."
Lee: "We should work on technologies that can do things that a person cannot do or cannot do well […] And don't just go for the bigger, faster, better algorithm, but think about the potential externalities [… that] come up with not just the electricity, but also the circuit breaker to make sure that the technology is safe and usable."
Sheffield: "Consider specializing in a domain application. There's so much richness in both our research and development and our contribution to the human society in the application of AI to a specific set of problems or a specific domain."
Clune: "AGI is coming soon. So, for researchers developing AI, I encourage you to work on techniques based on engineering, algorithms, meta-learning, end-to-end learning, etc., as those are most likely to be absorbed into the AGI that we're creating. For non-AI researchers, the most important are probably governance questions, so, What is the playbook for when AGI gets developed? Who gets to decide that playbook? And how do we get everyone on the planet to agree with that game plan?"
Rossi: "AI at this point is not just a science and a technology, it is a social technical discipline […] Start by talking with people that are not in your discipline so that together you can evaluate the impact of AI, of what you are working on, and be able to drive these technologies toward a future where scientific progress is supportive of human progress."
Marcus closed out the evening by recalling his statement in the last debate, "It takes a village to raise AI."
"I think that's even more true now," he said. "AI was a child before, now it's kind of like a rambunctious teenager, not yet completely in possession of mature judgment."
He concluded, "I think the moment is both exciting and perilous."