Why AI could be the key to turning the tide in the fight against cybercrime
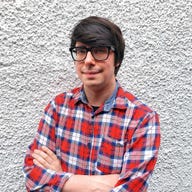
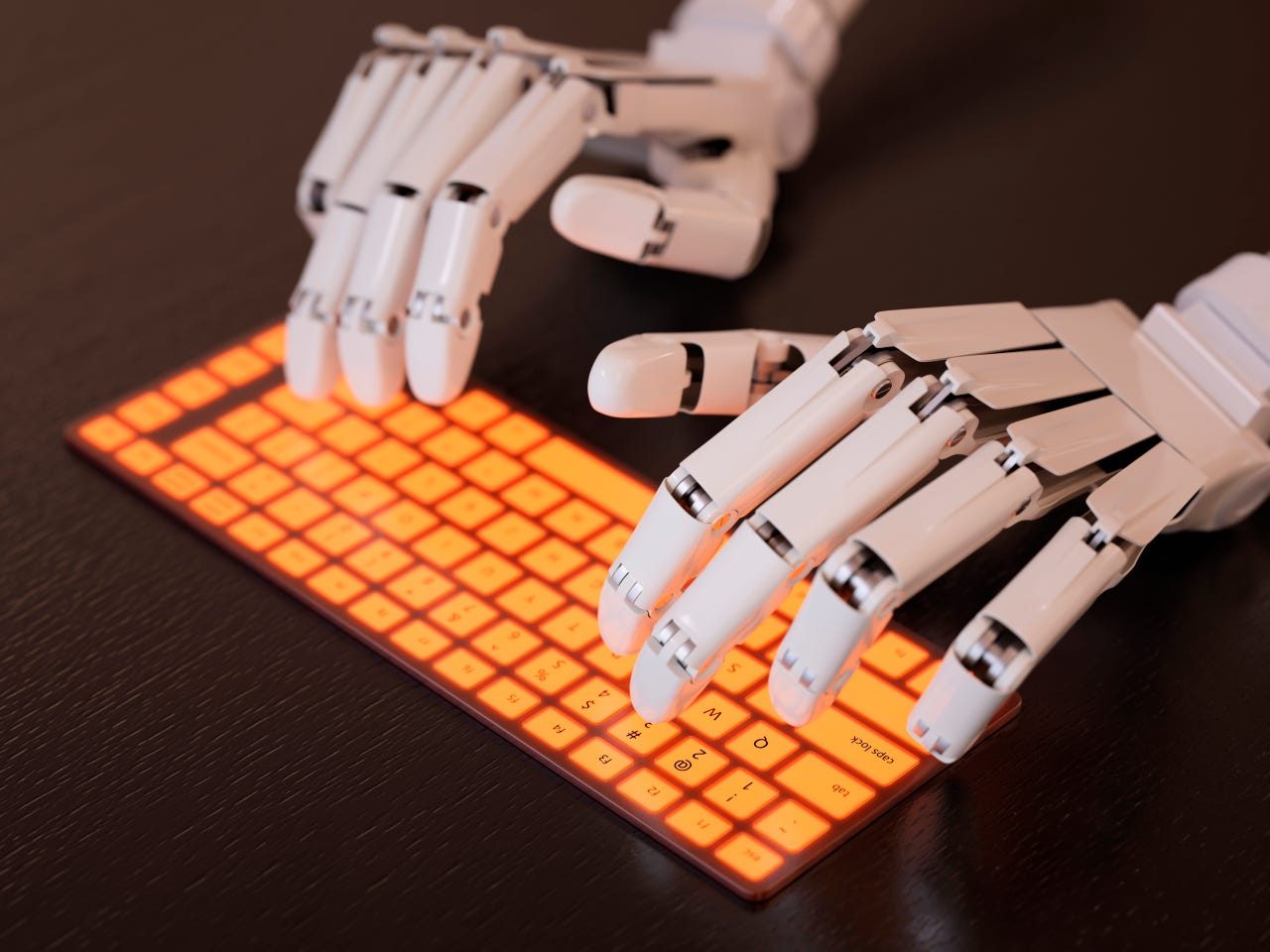
A specially programmed AI can 'think' about cybersecurity in a more complex detail than a human can.
It's not unreasonable to suggest the cybersecurity battle is being lost - and on more than one front.
Not only are more efficient and organised cybercriminals winning the security arms race against their corporate targets, there's also a shortage of cybersecurity professionals equipped with the skills required to fight hackers.
Some claim the fight against online crooks will be bolstered not by hiring more people but rather by machines using techniques based around artificial intelligence, machine learning, and deep learning.
This doesn't mean self-learning machines will be outright replacing cybersecurity professionals, however, but rather augmenting what they're able to do and taking care of the most basic tasks.
"We're not talking about any form of general artificial intelligence with cognitive capability, but a narrow AI with machine-learning capabilities," says Neil Thacker, deputy CISO at Forcepoint. He describes the security company's aims as "looking to use supervised learning so decision making doesn't actually require a human to make the decision".
Currently, cybersecurity operations, for the most part, require a human to spend their time going through alerts of potentially malicious activity -- a repetitive and time-consuming process, especially when you consider many will be false alarms.
"That's the human part, having to sift through lots of data," Thacker says. "Some of those alerts are benign but require a person to analyse the event itself, look at the potential consequences, and that's difficult to do".
And while this is boring for a human analyst, the more it analyses, the more AI can understand malware and fraudulent activity trends, which is something that will help cybersecurity professionals level the playing field in the fight against hackers.
"For cybersecurity, it definitely cuts down on the need for people to go through menial cases that are obvious false positives, because you can get more accurate and only show them things that are either suspected to be fraudulent or anomalies, which help the model learn best," says Stephen Whitworth, founder and data scientist at Ravelin Technology, a company founded by former Hailo staff, which deploys machine learning for fraud detection.
Ravelin believe that its machine-learning algorithm can do some things better than a person could, as the code is so specialised it can see things a human might miss.
"Machine learning allows you to think about things in a more complicated ways then a human can. If you think about a decision tree, there's more than 10 chained questions one after the other, and it's very hard for a human to encode this in their brain, whereas if you can have an algorithm which can do it, then it's much more efficient and can provide you with results you didn't have before," Whitworth says.
While there are a number of companies using machine learning to fight hacking and cybercrime, there are those who are already looking to take the technology even further with the use of deep learning. One of those is Israeli firm Deep Instinct, which lays claim to being the first company to apply deep learning to cybersecurity.
"With traditional machine learning, whenever you apply it, you need feature engineering, understand the features, then extract them. Or if you apply machine learning to malware detection, you find important features like APIs, interactions," says Dr Eli David, Deep Instinct's CTO and artificial intelligence expert.
Deep Instinct aims to detect previously-unknown malicious threats -- the sorts of attacks that might otherwise slip through the cracks, because they're too new to be noticed. "While we're good at detecting past threats, what we really care about is the detection of new threats," says Dr David.
Currently, he argues, it's simple for malicious software developers to enable their creations to evade detection, as slight modification of the code can make it unrecognisable. However, that can be made much more difficult with the introduction of deep learning.
"We're trying to make the detection rate as close as possible to 100 percent and make life as difficult as possible for creators of new lines of malware. Today, it's very easy; they modify a few lines of malware code and manage to evade detection by most solutions. But we hope to make life very difficult for them with detection rates of 99.99 percent," Dr David says.
So, if this deep learning technology is so good, why isn't it already mainstream? The answer is it's still very much something where "the barrier of entry is still extremely high," Dr David says. Unless you're of the likes of Google, Facebook, or Amazon, deep learning algorithms are "difficult to implement, hard to understand, and it's completely impossible if you don't have the GPU setup".
But given how quickly technology develops, and as the usage of machine learning and AI decline, these tools are likely to become pervasive.
"It's only going to get bigger and bigger. It's quicker. It's cheaper. It can think about fraudsters in ways as a human you wouldn't understand, and it can analyse tonnes more data being generated by hundreds of millions of people on mobile and on the internet," Whitworth says.
But Forcepoint's Thacker says there's a "big concern" about these techniques being used by the very people they're meant to be stopping.
"When you're looking at levels of automation over the next few years, it becomes about good versus bad. Who has the most trained models? Who has the most accurate and available data in order to learn in a structured learning environment?" he explains.
"Cybercriminals are going to be focusing on this area; it's cost effective for them to do this. So, we need to also be in that fight, and if we're behind and the attackers are ahead of us, we need to catch up with them," Thacker says.
However, there are those who remain unconvinced that artificial intelligence in cybersecurity is even yet a realistic concept. Eugene Kaspersky has spoken against the idea of it, and he argues that machine learning isn't a new phenomenon and shouldn't be counted as AI.
"So, is this machine learning AI? No. It's just computer algorithms -- in our case very good ones written with the highest grade of professionalism, talent, and passion for the fight against cyber-badness. To call it AI would be misleading at best, purposefully phony at worst," says Kaspersky.
"If someone does invent AI, as soon as it becomes known to the general public, it will have a colossal impact on a lot more besides the relatively small (albeit very important) cybersecurity field," he says.
Thacker seems to agree with Kaspersky on how some are overselling the potential of AI. "Some vendors talk about an AI capability without having a true capability yet," he says. However, he still sees a strong future for AI in cybersecurity because "it's one of the biggest pain points in organisations".
READ MORE ON CYBERSECURITY