Google AI can pinpoint where any snap was taken, just by looking at pixels

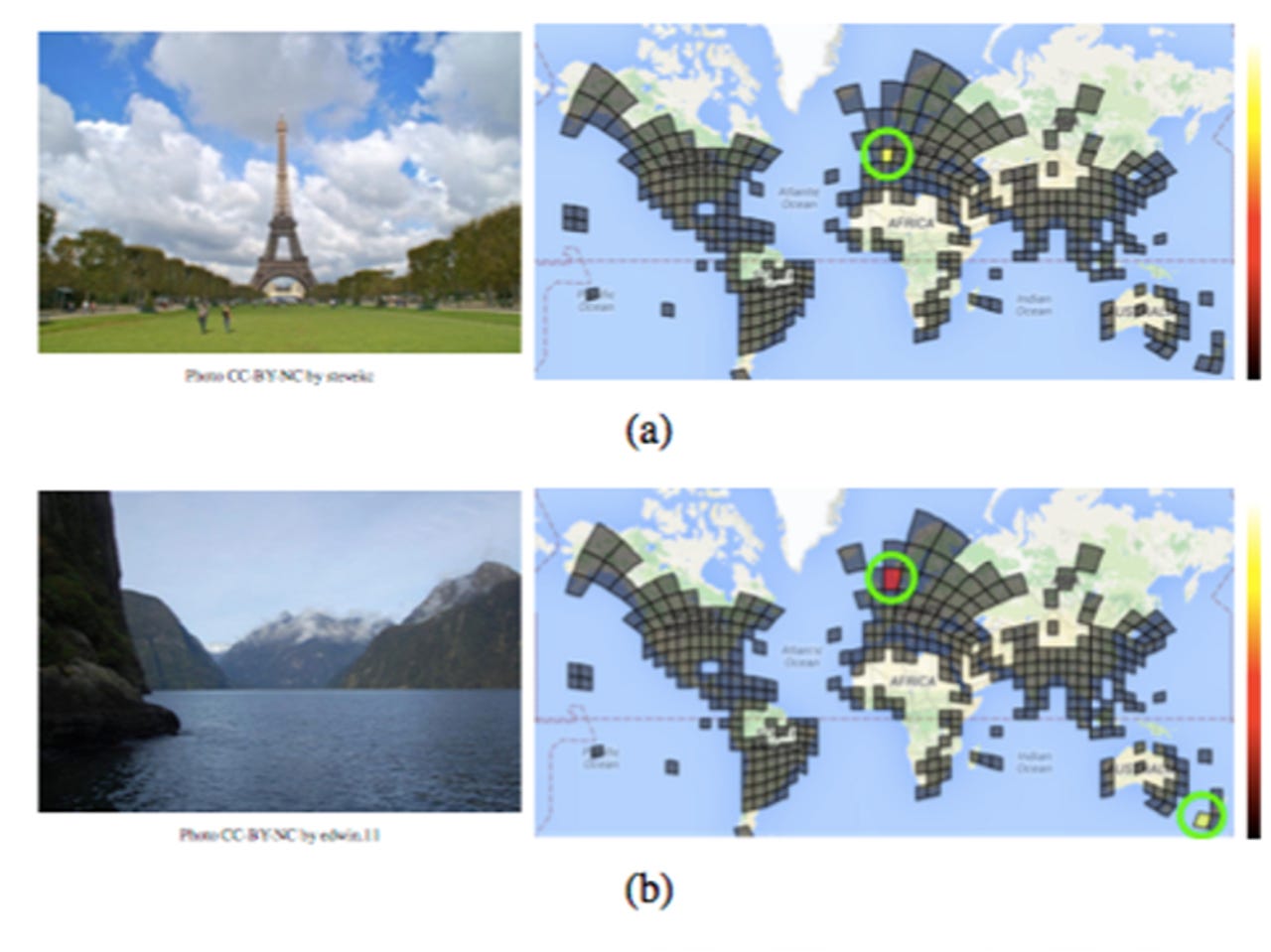
Google now has the ability to analyse any photo and pinpoint where in the world it was taken.
A new deep-learning machine from Google called PlaNet can outperform humans at identifying the location of a street scene or even indoor objects from an image.
Google now has the ability to analyse any photo and pinpoint where in the world it was taken. According to the location-guessing machine's creators, it can do that task with "superhuman levels of accuracy".
MIT Technology Review reports Google's PlaNet neural-network effort can perform this task only using an image's pixels.
The project is being led by Google computer-vision specialist Tobias Weyand, who in a new paper details how the researchers trained a convolutional neural network with a massive dataset of images sourced from Google+ with geotag Exif data or image metadata.
As the paper notes, past efforts have approached geolocation as an image-retrieval problem and have only been able to pick out landmarks to generate an approximate location.
PlaNet treats the task as a classification problem and uses multiple visual cues, including weather patterns, vegetation, road markings, and architectural details, to identify an exact location in some cases.
This approach allows it to "express uncertainty about a photo". So, for example, it can be fairly confident about the location of an image of the Eiffel Tower, whereas a picture of a fjord could be in New Zealand or Norway.
The system was developed by dividing the world into a grid of 26,000 squares. The more images taken in a given location, the bigger the square, so cities are larger than remote areas while oceans are completely ignored.
To train the network, Google used a dataset of 126 million images from the web with Exif image metadata, and then split off 91 million images for training and 34 million images for validation.
To see how PlaNet fared against 10 "well-travelled humans", the researchers then used the website Geoguessr and a collection of Street View images. PlaNet won 28 of 50 rounds, according to the paper.
Human subjects said they looked for "vegetation, the architectural style, the color of lane markings, and the direction of traffic on the street" -- and even had the benefit of ruling out China since Street View is not available there.
"One would expect that these cues, especially street signs, together with world knowledge and common sense, should give humans an unfair advantage over PlaNet, which was trained solely on image pixels and geolocations. Yet PlaNet was able to outperform humans by a considerable margin," the researchers boast.
To improve its geolocation abilities even further, for images that lack sufficient visual cues, the researchers trained the machine to do 'sequence location' -- that is, exploiting the way photos are often taken in sequences and so the system uses entire photo albums.
The training and testing data set in this instance was even larger. "For this task, we collected a dataset of 29.7 million public photo albums with geotags from Google+, which we split into 23.5 million training albums of 490 million images and 6.2 million testing albums of 126 million images," the researchers wrote.
This method proved even more effective at geolocation, with the researchers reporting 50 percent higher performance than the single-image model.